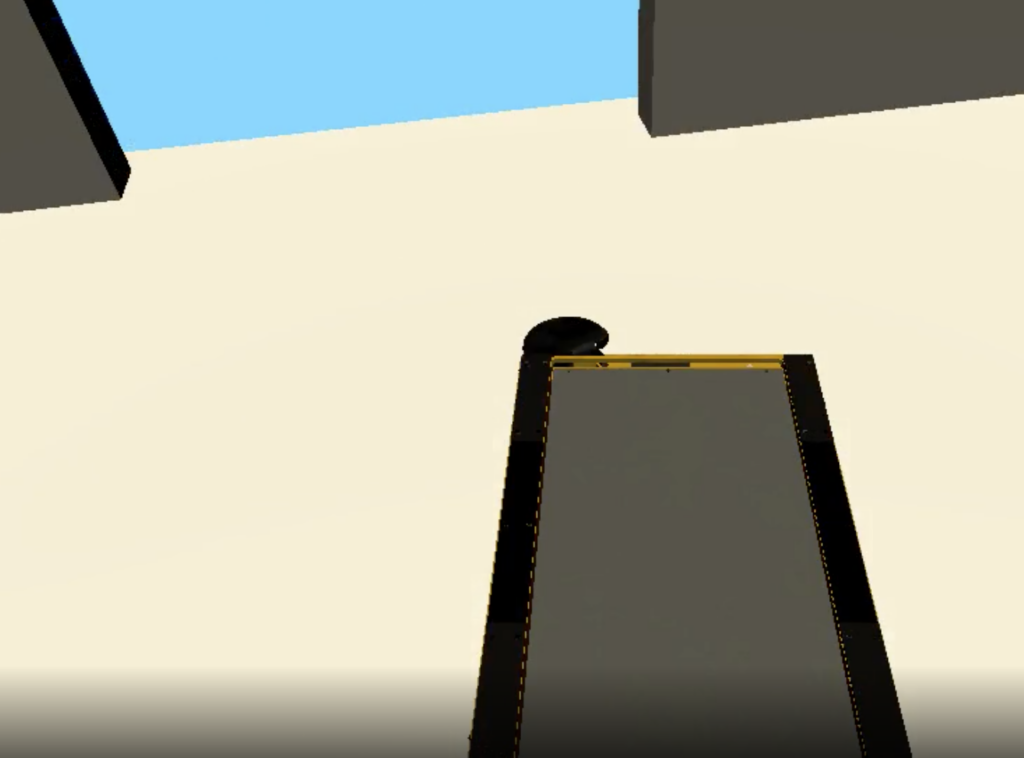
Deep Reinforcement Learning (DRL) has demonstrated great success in learning single or multiple tasks from scratch. Various DRL algorithms have been proposed and were applied in a broad class of tasks including chess and video games, robot navigation or robot manipulation.
In this work, we investigate the potential of DRL in a mapless navigation task within a warehouse. The challenges of the task are the partial observability of the space and the need of effective exploration strategies for fast learning of navigation strategies.
We trained a mobile robot (the agent) from scratch and compared how different sensor observations could influence the navigation performance. The evaluated sensors are a 360-degree Lidar sensor, only depth image and only RGB image. For Lidar and RGB inputs, we evaluated partial and full observability of the state space. We successfully trained the agent to navigate to a goal with a reward setting that is also applicable to the real world.
Currently, we are extending the work to multi-modal sensor inputs with both Lidar and RGB inputs (RGB image only frontal view) and incorporated self-curriculum learning on a more challenging navigation task in a warehouse and obtained promising initial outcomes.
The video shows learned navigation strategies using a single RGB-D camera mounted at the front of the robot. The results were obtained after 85.000 interactions (single action excution, e.g. wheel velocity commands).
This video shows the learnded strategy after 140.000 interactions with the environment.