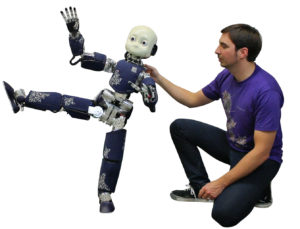
This lecture provides a unique overview over central topics in Cyber-Physical-Systems:
- Kinematics, Dynamics & Simulation of CPS
- Data Representations & Model Learning
- Feedback Control, Priorities & Torque Control
- Planning & Cognitive Reasoning
- Reinforcement Learning & Policy Search
The course provides a structured and well motivated overview over modern techniques and tools which enable the students to define learning problems in Cyber-Physical-Systems.
Links and Resources
Slides
- 03.102022 10:15 HS TPT Org & Intro (L1)
- 10.10.2022 10:15 CPS Lab Live Demos at CPS 10.10.22
- 17.10.2022 10:15 HS TPT Robot Kinematics Part I
- 17.10.2022 11:00 Study Center HS1 Digital Competences
- 24.10.2022 10:15 HS TPT Robot Kinematics Part II
- 10.11.2022 15:15 HS TPT Motion Representation Learning
- 17.11.2022 15:15 HS TPT Motion Representation Learning + Jupyter NB on Motion Representations
- 24.11.2022 15:15 HS TPT Probabilistic Motion Representations
- 13.01.2023 10:15 Online Feedback Control + Jupyter NB on PID Control
- 16.01.2023 10:15 HS TPT Reinforcement Learning & Markov Processes
- 27.01.2023 10:15 Online Exam Content & Questions
Exam Preparation & Q&A’s
- 13.01.2023 10:15 [Online only] Discussion of student questions regarding the exam. https://live.ai-lab.science/
- 20.01.2023 10:15 [Online only] Course Feedback & Discussion of student questions regarding the exam. https://live.ai-lab.science/
- 27.01.2023 10:15 [Online only] Discussion of student questions regarding the exam. https://live.ai-lab.science/
- 30.01.2023 10:15 HS Thermoprozesstechnik Written Exam
- 06.03.2023 10:15 Location not fixed Written Exam (2nd option)
Last Year’s Slides:
- Slides on Python (L2) 10.10.22
- Python Code Basics (L2) 17.10.22 [Python Code]
- Kinematics, Dynamics & Simulation (L3) 24.10.22 (CodeWithMe Link)[Python Code]
- Data Representations & Learning (L4) 31.10.22 (Instructions of Howto use CoppeliaSim with Python)
- Feedback Control (L5) 10.11.22
- Planning (L6) 17.11.22
- Reinforcement Learning I (L7) 24.11.2021
- Reinforcement Learning II (L8) 12.12.22
- Current Trends in Robotics (L9) 09.01.23
- Exam Preparation (L10) 16.01.23
- Content and Exam Q&A (L11) 23.01.23
- Written Exam 30.01.23
Exam Dates
- 30.01.2023 at 10:15 in HS Thermoprozesstechnik.
- 06.03.2023 at 10:15 in HS Thermoprozesstechnik.
- upon request via cps@unileoben.ac.at.
Location & Time
- Location: HS Thermoprozesstechnik
- Dates: Mondays, 10:15 – 12:00
Learning objectives / qualifications
- Students get a comprehensive understanding of Cyber-Physical-Systems.
- Students learn to analyze the challenges in simulating, modeling and controlling CPS.
- Students understand and can apply basic machine learning and control techniques in CPS.
- Students know how to analyze the models’ results, improve the model parameters and can interpret the model predictions and their relevance.
Programming Assignments & Simulation Tools
For simulating robotic systems, we will use the tool CoppeliaSim. The tool can be used for free for research and for teaching.
To experiment with state of the art robot control and learning methods Python will be used. If you never used Python and are unexperienced in programming, please visit the tutorials on Python programming prior to the lecture.
The course will also use the tool Code With Me from JetBrains. With this stool, we can develop jointly code.
Literature
- The Probabilistic Machine Learning book by Univ.-Prof. Dr. Elmar Rueckert.
- Bishop 2006. Pattern Recognition and Machine Learning, Springer.
- Barber 2007. Bayesian Reasoning and Machine Learning, Cambridge University Press.
- Murray, Li and Sastry 1994. A mathematical introduction to robotic manipulation, CRC Press.
- B. Siciliano, L. Sciavicco 2009. Robotics: Modelling,Planning and Control, Springer.
- Kevin M. Lynch and Frank C. Park 2017. MODERN ROBOTICS, MECHANICS, PLANNING, AND CONTROL, Cambridge University Press.