Author: Elmar Rueckert
Beschaffung SAP Prozessablauf
MATLAB Code of Probabilistic Movement Primitives for Motion Analysis
Matlab Code Link
Publication where the Code was used
2016 |
|
Rueckert, Elmar; Camernik, Jernej; Peters, Jan; Babic, Jan Probabilistic Movement Models Show that Postural Control Precedes and Predicts Volitional Motor Control Journal Article In: Nature Publishing Group: Scientific Reports, vol. 6, no. 28455, 2016. @article{Rueckert2016b, | ![]() |
MATLAB Code of Spiking Neural Networks for Robot Motion Planning
Matlab Code Link
Publication where the Code was used
2016 |
|
Rueckert, Elmar; Kappel, David; Tanneberg, Daniel; Pecevski, Dejan; Peters, Jan Recurrent Spiking Networks Solve Planning Tasks Journal Article In: Nature Publishing Group: Scientific Reports, vol. 6, no. 21142, 2016. @article{Rueckert2016a, | ![]() |
Stochastic Neural Networks for Robot Motion Planning
Video
Link to the file
You may use this video for research and teaching purposes. Please cite the Chair of Cyber-Physical-Systems or the corresponding research paper.
Publications
2016 |
|
Tanneberg, Daniel; Paraschos, Alexandros; Peters, Jan; Rueckert, Elmar Deep Spiking Networks for Model-based Planning in Humanoids Proceedings Article In: Proceedings of the International Conference on Humanoid Robots (HUMANOIDS), 2016. @inproceedings{tanneberg_humanoids16, | ![]() |
Rueckert, Elmar; Kappel, David; Tanneberg, Daniel; Pecevski, Dejan; Peters, Jan Recurrent Spiking Networks Solve Planning Tasks Journal Article In: Nature Publishing Group: Scientific Reports, vol. 6, no. 21142, 2016. @article{Rueckert2016a, | ![]() |
Learning Bimanual Manipulation Primitives
Video
Link to the file
You may use this video for research and teaching purposes. Please cite the Chair of Cyber-Physical-Systems or the corresponding research paper.
Learning Multimodal Solutions with Movement Primitives
Video
Link to the file
You may use this video for research and teaching purposes. Please cite the Chair of Cyber-Physical-Systems or the corresponding research paper.
Publications
2015 |
|
Rueckert, Elmar; Mundo, Jan; Paraschos, Alexandros; Peters, Jan; Neumann, Gerhard Extracting Low-Dimensional Control Variables for Movement Primitives Proceedings Article In: Proceedings of the International Conference on Robotics and Automation (ICRA), 2015. @inproceedings{Rueckert2015, | ![]() |
Dynamic Control of a CableBot
Building a CableBot and Learning the Dynamics Model and the Controller
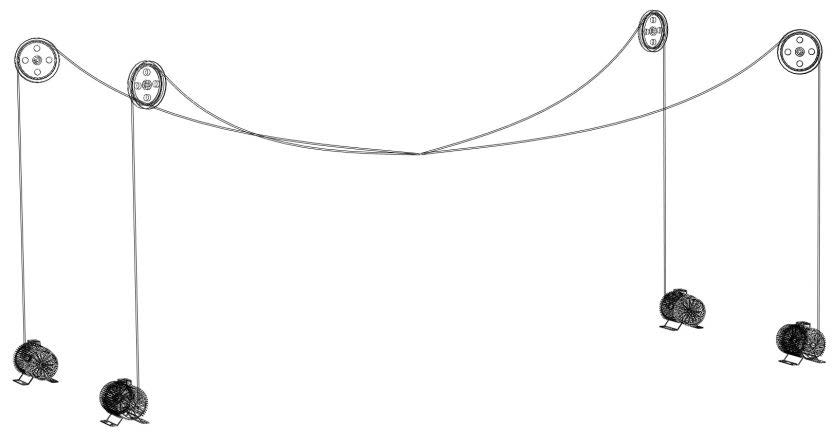
Controlling cable driven master slave robots is a challenging task. Fast and precise motion planning requires stabilizing struts which are disruptive elements in robot-assisted surgeries. In this work, we study parallel kinematics with an active deceleration mechanism that does not require any hindering struts for stabilization.
Reinforcement learning is used to learn control gains and model parameters which allow for fast and precise robot motions without overshooting. The developed mechanical design as well as the controller optimization framework through learning can improve the motion and tracking performance of many widely used cable-driven master slave robots in surgical robotics.
Project Consortium
- Montanuniversität Leoben
Related Work
H Yuan, E Courteille, D Deblaise (2015). Static and dynamic stiffness analyses of cable-driven parallel robots with non-negligible cable mass and elasticity, Mechanism and Machine Theory, 2015 – Elsevier, link.
MA Khosravi, HD Taghirad (2011). Dynamic analysis and control of cable driven robots with elastic cables, Transactions of the Canadian Society for Mechanical Engineering 35.4 (2011): 543-557, link.
Publications
2019 |
|
Rueckert, Elmar; Jauer, Philipp; Derksen, Alexander; Schweikard, Achim Dynamic Control Strategies for Cable-Driven Master Slave Robots Proceedings Article In: Keck, Tobias (Ed.): Proceedings on Minimally Invasive Surgery, Luebeck, Germany, 2019, (January 24-25, 2019). @inproceedings{Rueckert2019c, | ![]() |
Active transfer learning with neural networks through human-robot interactions (TRAIN)
DFG Project 07/2020-01/2025
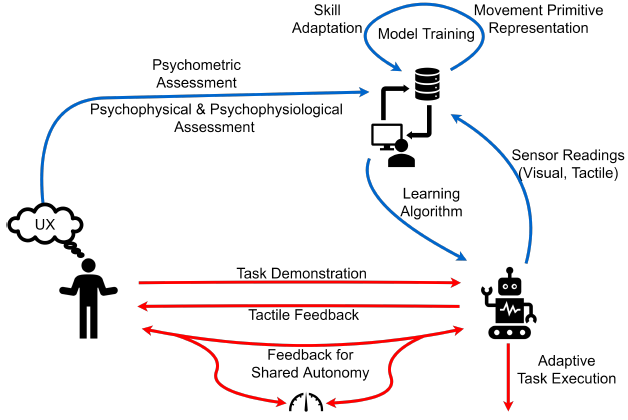
In our vision, autonomous robots are interacting with humans at industrial sites, in health care, or at our homes managing the household. From a technical perspective, all these application domains require that robots process large amounts of data of noisy sensor observations during the execution of thousands of different motor and manipulation skills. From the perspective of many users, programming these skills manually or using recent learning approaches, which are mostly operable only by experts, will not be feasible to use intelligent autonomous systems in tasks of everyday life.
In this project, we aim at improving robot skill learning with deep networks considering human feedback and guidance. The human teacher is rating different transfer learning strategies in the artificial neural network to improve the learning of novel skills by optimally exploiting existing encoded knowledge. Neural networks are ideally suited for this task as we can gradually increase the number of transferred parameters and can even transition between the transfer of task specific knowledge to abstract features encoded in deeper layers. To consider this systematically, we evaluate subjective feedback and physiological data from user experiments and elaborate assessment criteria that enable the development of human-oriented transfer learning methods. In two main experiments, we first investigate how users experience transfer learning and then examine the influence of shared autonomy of humans and robots. This will result in a methodical robot skill learning framework that adapts to the users’ needs, e.g., by adjusting the degree of autonomy of the robot to laymen requirements. Even though we evaluate the learning framework focusing on pick and place tasks with anthropomorphic robot arms, our results will be transferable to a broad range of human-robot interaction scenarios including collaborative manipulation tasks in production and assembly, but also for designing advanced controls for rehabilitation and household robots.
Project Consortium
Friedrich-Alexander-Universität Erlangen-Nürnberg
- Montanuniversität Leoben
Links
Details on the research project can be found on the project webpage.
Publications
2021 |
|
Tanneberg, Daniel; Ploeger, Kai; Rueckert, Elmar; Peters, Jan SKID RAW: Skill Discovery from Raw Trajectories Journal Article In: IEEE Robotics and Automation Letters (RA-L), pp. 1–8, 2021, ISSN: 2377-3766, (© 20XX IEEE. Personal use of this material is permitted. Permission from IEEE must be obtained for all other uses, in any current or future media, including reprinting/republishing this material for advertising or promotional purposes, creating new collective works, for resale or redistribution to servers or lists, or reuse of any copyrighted component of this work in other works.). @article{Tanneberg2021, | ![]() |
Jamsek, Marko; Kunavar, Tjasa; Bobek, Urban; Rueckert, Elmar; Babic, Jan Predictive exoskeleton control for arm-motion augmentation based on probabilistic movement primitives combined with a flow controller Journal Article In: IEEE Robotics and Automation Letters (RA-L), pp. 1–8, 2021, ISSN: 2377-3766, (© 20XX IEEE. Personal use of this material is permitted. Permission from IEEE must be obtained for all other uses, in any current or future media, including reprinting/republishing this material for advertising or promotional purposes, creating new collective works, for resale or redistribution to servers or lists, or reuse of any copyrighted component of this work in other works.). @article{Jamsek2021, | ![]() |
Cansev, Mehmet Ege; Xue, Honghu; Rottmann, Nils; Bliek, Adna; Miller, Luke E.; Rueckert, Elmar; Beckerle, Philipp Interactive Human-Robot Skill Transfer: A Review of Learning Methods and User Experience Journal Article In: Advanced Intelligent Systems, pp. 1–28, 2021. @article{Cansev2021, | ![]() |
2020 |
|
Rottmann, N.; Kunavar, T.; Babič, J.; Peters, J.; Rueckert, E. Learning Hierarchical Acquisition Functions for Bayesian Optimization Proceedings Article In: International Conference on Intelligent Robots and Systems (IROS’ 2020), 2020. @inproceedings{Rottmann2020HiBO, | ![]() |
Xue, H.; Boettger, S.; Rottmann, N.; Pandya, H.; Bruder, R.; Neumann, G.; Schweikard, A.; Rueckert, E. Sample-Efficient Covariance Matrix Adaptation Evolutional Strategy via Simulated Rollouts in Neural Networks Proceedings Article In: International Conference on Advances in Signal Processing and Artificial Intelligence (ASPAI’ 2020), 2020. @inproceedings{Xue2020, | ![]() |