Ph.D. Student at the University of Luebeck
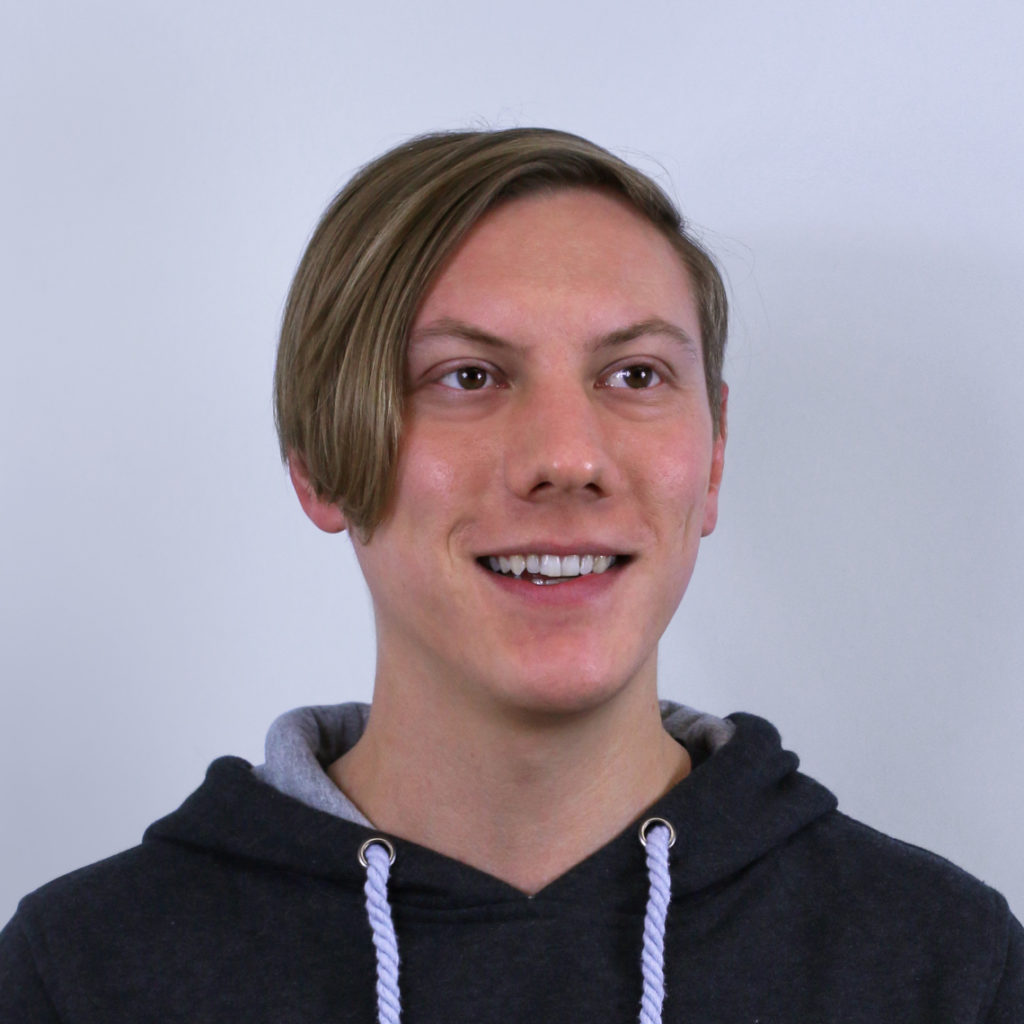
Short bio: Dr. Daniel Tanneberg passed his PhD defense on the 3rd of December in 2020. He is now working as senior researcher at the Honda Research Institute in Offenbach, Germany.
He was co-supervised by Prof. Jan Peters from the Technische Universitaet Darmstadt and Univ.-Prof. Dr. Elmar Rueckert, the head of this lab.
Daniel has joined the Intelligent Autonomous Systems (IAS) Group at the Technische Universitaet Darmstadt in October 2015 as a Ph.D. Student. His research focused on (biologically-inspired) machine learning for robotics and neuroscience. During his Ph.D., Daniel investigated the applicability and properties of spiking and memory-augmented deep neural networks. His neural networks were applied to robotic as well as to algorithmic tasks.
With his masters thesis with the title Neural Networks Solve Robot Planning Problems he won the prestigoues Hanns-Voith-Stiftungspreis 2017 ’Digital Solutions’.
Research Interests
- (Biologically-inspired) Machine Learning, (Memory-augmented) Neural Networks, Deep Learning, (Stochastic) Neural Networks, Lifelong-Learning.
Contact & Quick Links
Dr. Daniel Tanneberg
Former Doctoral Student supervised by Prof. Dr. Jan Peters and Univ.-Prof. Dr. Elmar Rueckert from 10/2015 to 12/2020.
Hochschulstr. 10,
64289 Darmstadt,
Deutschland
Email: daniel@robot-learning.de
Web: https://www.rob.uni-luebeck.de/index.php?id=460
Publcations
2021 |
|
Tanneberg, Daniel; Ploeger, Kai; Rueckert, Elmar; Peters, Jan SKID RAW: Skill Discovery from Raw Trajectories Journal Article In: IEEE Robotics and Automation Letters (RA-L), pp. 1–8, 2021, ISSN: 2377-3766, (© 20XX IEEE. Personal use of this material is permitted. Permission from IEEE must be obtained for all other uses, in any current or future media, including reprinting/republishing this material for advertising or promotional purposes, creating new collective works, for resale or redistribution to servers or lists, or reuse of any copyrighted component of this work in other works.). @article{Tanneberg2021, | ![]() |
2020 |
|
Tanneberg, Daniel; Rueckert, Elmar; Peters, Jan Evolutionary training and abstraction yields algorithmic generalization of neural computers Journal Article In: Nature Machine Intelligence, pp. 1–11, 2020. @article{Tanneberg2020, | ![]() |
2019 |
|
Tanneberg, Daniel; Peters, Jan; Rueckert, Elmar Intrinsic Motivation and Mental Replay enable Efficient Online Adaptation in Stochastic Recurrent Networks Journal Article In: Neural Networks – Elsevier, vol. 109, pp. 67-80, 2019, ISBN: 0893-6080, (Impact Factor of 7.197 (2017)). @article{Tanneberg2019, | ![]() |
2017 |
|
Tanneberg, Daniel; Peters, Jan; Rueckert, Elmar Efficient Online Adaptation with Stochastic Recurrent Neural Networks Proceedings Article In: Proceedings of the International Conference on Humanoid Robots (HUMANOIDS), 2017. @inproceedings{Tanneberg2017a, | ![]() |
Thiem, Simon; Stark, Svenja; Tanneberg, Daniel; Peters, Jan; Rueckert, Elmar Simulation of the underactuated Sake Robotics Gripper in V-REP Proceedings Article In: Workshop at the International Conference on Humanoid Robots (HUMANOIDS), 2017. @inproceedings{Thiem2017b, | ![]() |
Tanneberg, Daniel; Peters, Jan; Rueckert, Elmar Online Learning with Stochastic Recurrent Neural Networks using Intrinsic Motivation Signals Proceedings Article In: Proceedings of the Conference on Robot Learning (CoRL), 2017. @inproceedings{Tanneberg2017, | ![]() |
2016 |
|
Tanneberg, Daniel; Paraschos, Alexandros; Peters, Jan; Rueckert, Elmar Deep Spiking Networks for Model-based Planning in Humanoids Proceedings Article In: Proceedings of the International Conference on Humanoid Robots (HUMANOIDS), 2016. @inproceedings{tanneberg_humanoids16, | ![]() |
Rueckert, Elmar; Kappel, David; Tanneberg, Daniel; Pecevski, Dejan; Peters, Jan Recurrent Spiking Networks Solve Planning Tasks Journal Article In: Nature Publishing Group: Scientific Reports, vol. 6, no. 21142, 2016. @article{Rueckert2016a, | ![]() |
Sharma, David; Tanneberg, Daniel; Grosse-Wentrup, Moritz; Peters, Jan; Rueckert, Elmar Adaptive Training Strategies for BCIs Proceedings Article In: Cybathlon Symposium, 2016. @inproceedings{Sharma2016, | ![]() |