2025
|
Neubauer, Melanie; Özdenizci, Ozan; Piater, Justus; Rueckert, Elmar Sparsifying instance segmentation models for efficient vision-based industrial recycling Proceedings Article Forthcoming In: European Conference on Machine Learning and Principles and Practice of Knowledge Discovery in Databases, Forthcoming. @inproceedings{neubauer2025ibis,
title = {Sparsifying instance segmentation models for efficient vision-based industrial recycling},
author = {Melanie Neubauer and Ozan Özdenizci and Justus Piater and Elmar Rueckert },
url = {https://cloud.cps.unileoben.ac.at/index.php/s/RzZNxYmJbTN8fCb},
year = {2025},
date = {2025-09-15},
booktitle = {European Conference on Machine Learning and Principles and Practice of Knowledge Discovery in Databases},
keywords = {},
pubstate = {forthcoming},
tppubtype = {inproceedings}
}
| |
Vanjani, Pankhuri; Mattes, Paul; Li, Maximilian Xiling; Dave, Vedant; Lioutikov, Rudolf DisDP: Robust Imitation Learning via Disentangled Diffusion Policies Proceedings Article Forthcoming In: Reinforcement Learning Conference (RLC), Reinforcement Learning Journal, Forthcoming. @inproceedings{dave2025disdp,
title = {DisDP: Robust Imitation Learning via Disentangled Diffusion Policies},
author = {Pankhuri Vanjani and Paul Mattes and Maximilian Xiling Li and Vedant Dave and Rudolf Lioutikov},
year = {2025},
date = {2025-05-09},
urldate = {2025-05-09},
booktitle = {Reinforcement Learning Conference (RLC)},
publisher = {Reinforcement Learning Journal},
keywords = {Contrastive Learning, Imitation Learning, Representation Learning},
pubstate = {forthcoming},
tppubtype = {inproceedings}
}
| 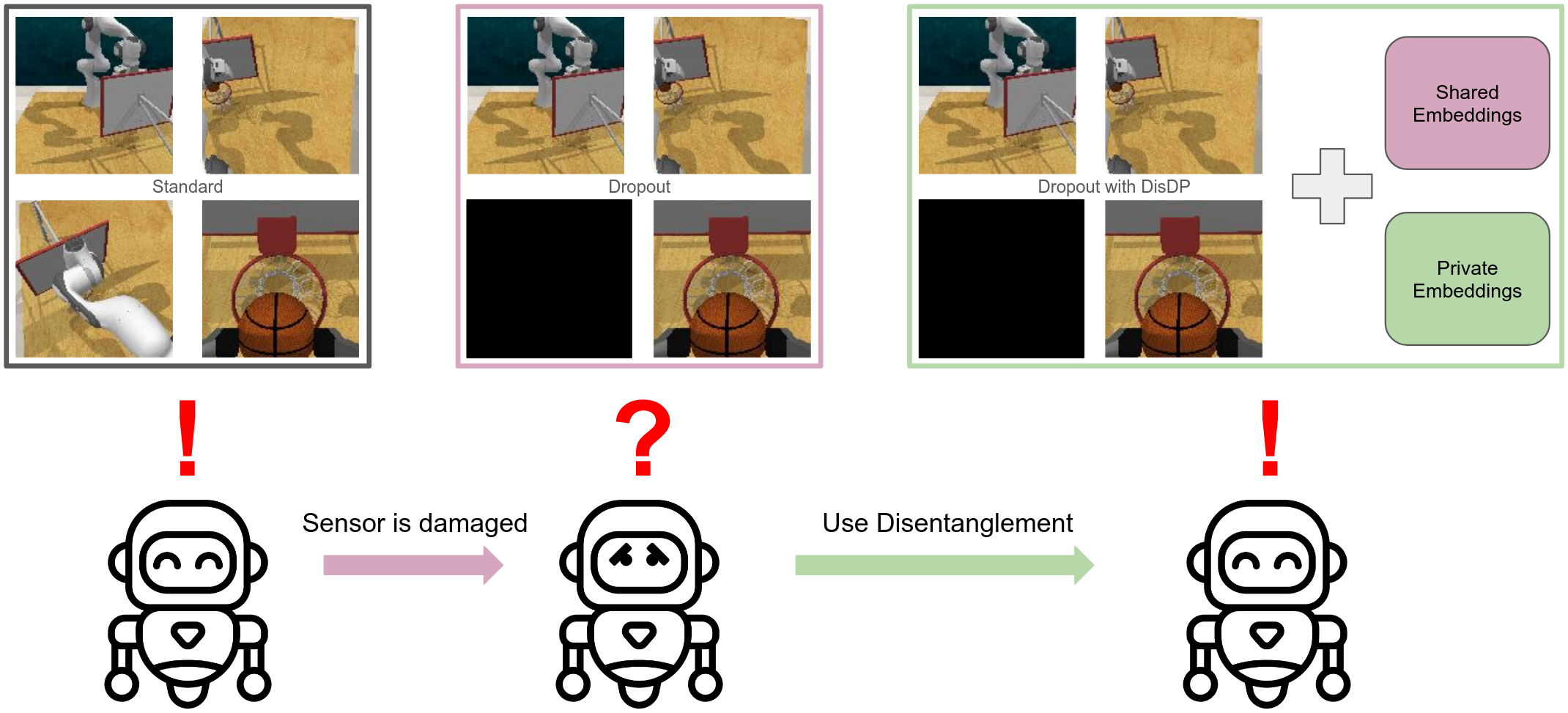 |
Dave, Vedant; Rueckert, Elmar Skill Disentanglement in Reproducing Kernel Hilbert Space Proceedings Article In: Proceedings of the AAAI Conference on Artificial Intelligence (AAAI), pp. 16153-16162, 2025. @inproceedings{Dave2025bb,
title = {Skill Disentanglement in Reproducing Kernel Hilbert Space},
author = {Vedant Dave and Elmar Rueckert
},
url = {https://cloud.cps.unileoben.ac.at/index.php/s/m9XKo4t2FXAH6Cs},
doi = {https://doi.org/10.1609/aaai.v39i15.33774},
year = {2025},
date = {2025-04-11},
urldate = {2025-02-27},
booktitle = {Proceedings of the AAAI Conference on Artificial Intelligence (AAAI)},
volume = {39},
number = {15},
pages = {16153-16162},
abstract = {Unsupervised Skill Discovery aims at learning diverse skills without any extrinsic rewards and leverage them as prior for learning a variety of downstream tasks. Existing approaches to unsupervised reinforcement learning typically involve discovering skills through empowerment-driven techniques or by maximizing entropy to encourage exploration. However, this mutual information objective often results in either static skills that discourage exploration or maximise coverage at the expense of non-discriminable skills. Instead of focusing only on maximizing bounds on f-divergence, we combine it with Integral Probability Metrics to maximize the distance between distributions to promote behavioural diversity and enforce disentanglement. Our method, Hilbert Unsupervised Skill Discovery (HUSD), provides an additional objective that seeks to obtain exploration and separability of state-skill pairs by maximizing the Maximum Mean Discrepancy between the joint distribution of skills and states and the product of their marginals in Reproducing Kernel Hilbert Space. Our results on Unsupervised RL Benchmark show that HUSD outperforms previous exploration algorithms on state-based tasks.},
keywords = {Deep Learning, neural network, Reinforcement Learning, Skill Discovery, Unsupervised Learning},
pubstate = {published},
tppubtype = {inproceedings}
}
Unsupervised Skill Discovery aims at learning diverse skills without any extrinsic rewards and leverage them as prior for learning a variety of downstream tasks. Existing approaches to unsupervised reinforcement learning typically involve discovering skills through empowerment-driven techniques or by maximizing entropy to encourage exploration. However, this mutual information objective often results in either static skills that discourage exploration or maximise coverage at the expense of non-discriminable skills. Instead of focusing only on maximizing bounds on f-divergence, we combine it with Integral Probability Metrics to maximize the distance between distributions to promote behavioural diversity and enforce disentanglement. Our method, Hilbert Unsupervised Skill Discovery (HUSD), provides an additional objective that seeks to obtain exploration and separability of state-skill pairs by maximizing the Maximum Mean Discrepancy between the joint distribution of skills and states and the product of their marginals in Reproducing Kernel Hilbert Space. Our results on Unsupervised RL Benchmark show that HUSD outperforms previous exploration algorithms on state-based tasks. | 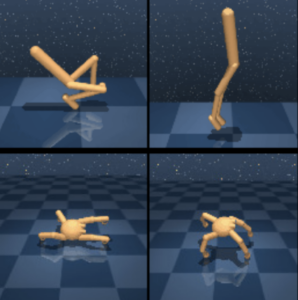 |
Nwankwo, Linus; Ellensohn, Bjoern; Dave, Vedant; Hofer, Peter; Forstner, Jan; Villneuve, Marlene; Galler, Robert; Rueckert, Elmar EnvoDat: A Large-Scale Multisensory Dataset for Robotic Spatial Awareness and Semantic Reasoning in Heterogeneous Environments Proceedings Article In: IEEE International Conference on Robotics and Automation (ICRA 2025)., 2025. @inproceedings{Nwankwo2025,
title = {EnvoDat: A Large-Scale Multisensory Dataset for Robotic Spatial Awareness and Semantic Reasoning in Heterogeneous Environments},
author = {Linus Nwankwo and Bjoern Ellensohn and Vedant Dave and Peter Hofer and Jan Forstner and Marlene Villneuve and Robert Galler and Elmar Rueckert},
url = {https://cloud.cps.unileoben.ac.at/index.php/s/MawgtYSbTBoNBZo},
year = {2025},
date = {2025-01-27},
urldate = {2025-01-27},
booktitle = {IEEE International Conference on Robotics and Automation (ICRA 2025).},
keywords = {Autonomous Navigation, robotics, SLAM},
pubstate = {published},
tppubtype = {inproceedings}
}
| 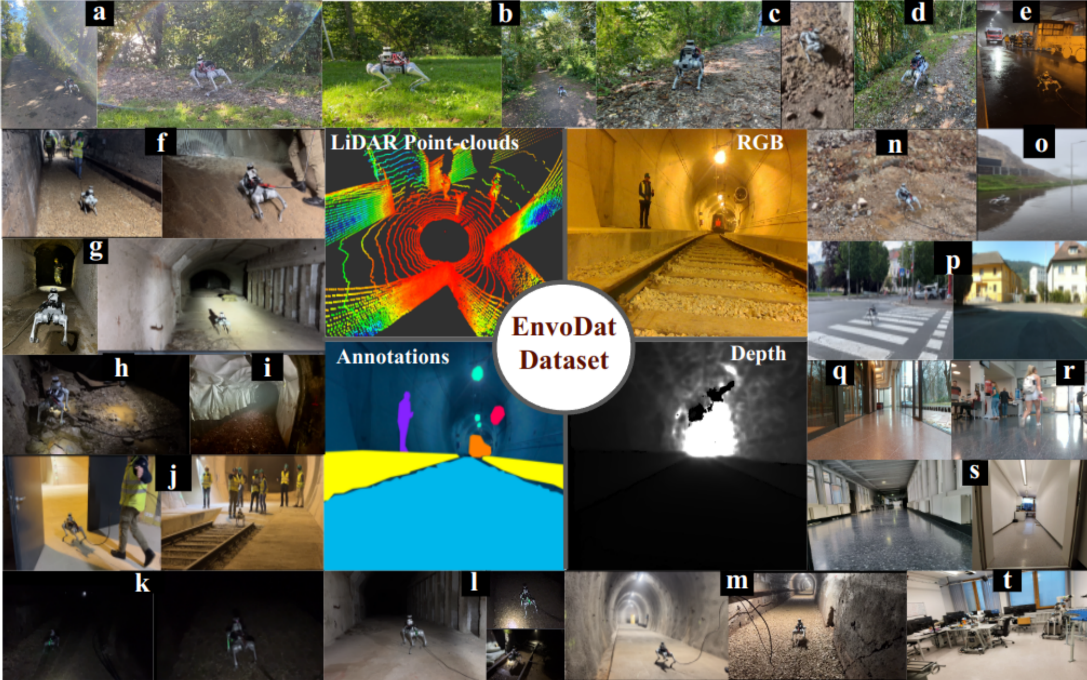 |
Oezdenizci, Ozan; Rueckert, Elmar; Legenstein, Robert Privacy-Aware Lifelong Learning Proceedings Article In: International Conference on Learning Representations (ICLR), 2025. @inproceedings{Oezdenizci2025,
title = {Privacy-Aware Lifelong Learning},
author = {Ozan Oezdenizci and Elmar Rueckert and Robert Legenstein},
url = {https://cloud.cps.unileoben.ac.at/index.php/s/4GLWiwFrYfZyFyS},
year = {2025},
date = {2025-01-22},
urldate = {2025-01-22},
booktitle = {International Conference on Learning Representations (ICLR)},
keywords = {Deep Learning, machine learning},
pubstate = {published},
tppubtype = {inproceedings}
}
| 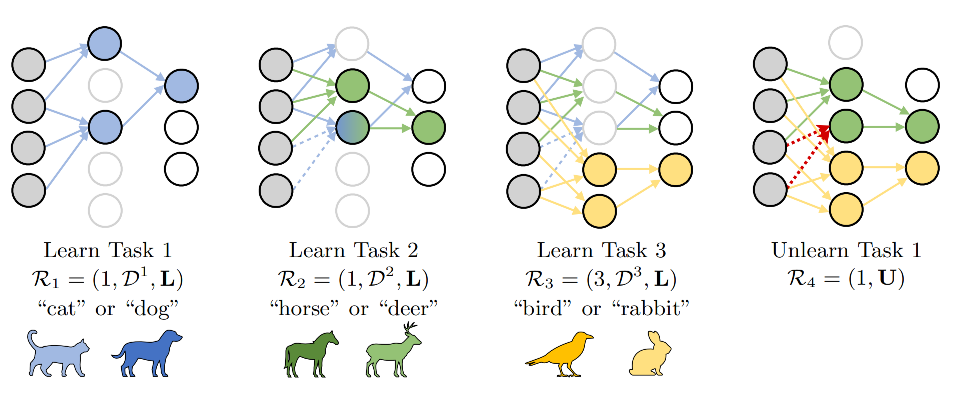 |
2024
|
Krukenfellner, Philip; Rueckert, Elmar; Flachberger, Helmut Predicting condition states, based on displacement data, generated by acceleration sensors on industrial linear vibrating screens through neural networks Journal Article In: IEEE Sensors Journal, pp. 1–13, 2024, ISBN: 1558-1748. @article{Krukenfellner2024,
title = {Predicting condition states, based on displacement data, generated by acceleration sensors on industrial linear vibrating screens through neural networks},
author = {Philip Krukenfellner and Elmar Rueckert and Helmut Flachberger},
url = {https://cloud.cps.unileoben.ac.at/index.php/s/GJN9XCBzW5TqPys},
isbn = {1558-1748},
year = {2024},
date = {2024-10-04},
urldate = {2024-10-04},
journal = {IEEE Sensors Journal},
pages = {1--13},
keywords = {Applied Deep Learning, Industrial Applications, Vibrating Screens},
pubstate = {published},
tppubtype = {article}
}
| 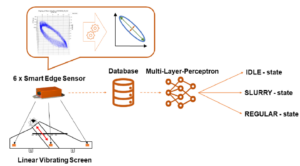 |
Dave, Vedant; Rueckert, Elmar Denoised Predictive Imagination: An Information-theoretic approach for learning World Models Workshop European Workshop on Reinforcement Learning (EWRL), 2024. @workshop{dpidave2024,
title = {Denoised Predictive Imagination: An Information-theoretic approach for learning World Models},
author = {Vedant Dave and Elmar Rueckert},
url = {https://cps.unileoben.ac.at/135_denoised_predictive_imagin/},
year = {2024},
date = {2024-08-01},
urldate = {2024-08-01},
publisher = {European Workshop on Reinforcement Learning (EWRL)},
abstract = {Humans excel at isolating relevant information from noisy data to predict the behavior of dynamic systems, effectively disregarding non-informative, temporally-correlated noise. In contrast, existing reinforcement learning algorithms face challenges in generating noise-free predictions within high-dimensional, noise-saturated environments, especially when trained on world models featuring realistic background noise extracted from natural video streams. We propose a novel information-theoretic approach that learn world models based on minimising the past information and retaining maximal information about the future, aiming at simultaneously learning control policies and at producing denoised predictions. Utilizing Soft Actor-Critic agents augmented with an information-theoretic auxiliary loss, we validate our method's effectiveness on complex variants of the standard DeepMind Control Suite tasks, where natural videos filled with intricate and task-irrelevant information serve as a background. Experimental results demonstrate that our model outperforms nine state-of-the-art approaches in various settings where natural videos serve as dynamic background noise. Our analysis also reveals that all these methods encounter challenges in more complex environments.},
keywords = {computer vision, Contrastive Learning, Reinforcement Learning, Representation Learning},
pubstate = {published},
tppubtype = {workshop}
}
Humans excel at isolating relevant information from noisy data to predict the behavior of dynamic systems, effectively disregarding non-informative, temporally-correlated noise. In contrast, existing reinforcement learning algorithms face challenges in generating noise-free predictions within high-dimensional, noise-saturated environments, especially when trained on world models featuring realistic background noise extracted from natural video streams. We propose a novel information-theoretic approach that learn world models based on minimising the past information and retaining maximal information about the future, aiming at simultaneously learning control policies and at producing denoised predictions. Utilizing Soft Actor-Critic agents augmented with an information-theoretic auxiliary loss, we validate our method's effectiveness on complex variants of the standard DeepMind Control Suite tasks, where natural videos filled with intricate and task-irrelevant information serve as a background. Experimental results demonstrate that our model outperforms nine state-of-the-art approaches in various settings where natural videos serve as dynamic background noise. Our analysis also reveals that all these methods encounter challenges in more complex environments. | 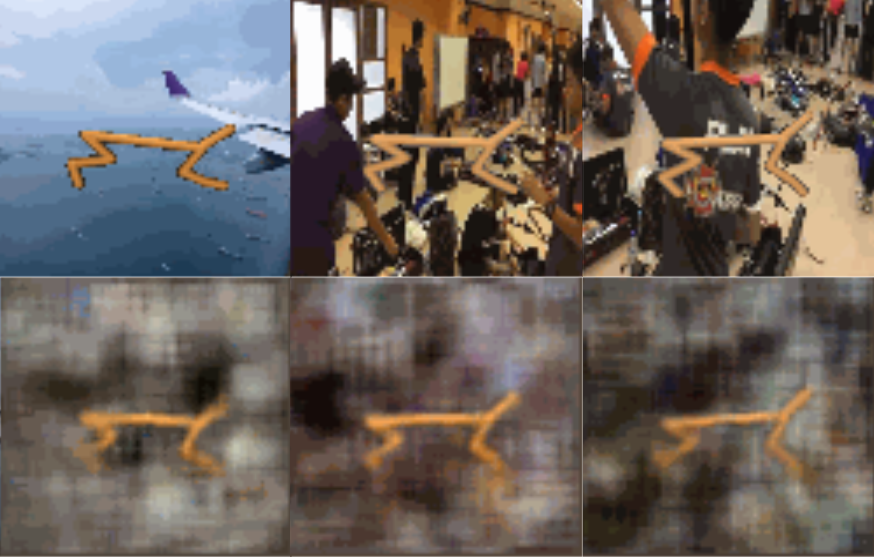 |
Trimmel, Simone; Spörl, Philipp; Haluza, Daniela; Lashin, Nagi; Meisel, Thomas C.; Pitha, Ulrike; Prohaska, Thomas; Puschenreiter, Markus; Rückert, Elmar; Spangl, Bernhard; Wiedenhofer, Dominik; Irrgeher, Johanna Green and blue infrastructure as model system for emissions of technology-critical elements Journal Article In: Science of The Total Environment, vol. 934, 2024, ISBN: 0048-9697, (https://doi.org/10.1016/j.scitotenv.2024.173364). @article{Trimmel2024,
title = {Green and blue infrastructure as model system for emissions of technology-critical elements},
author = {Simone Trimmel and Philipp Spörl and Daniela Haluza and Nagi Lashin and Thomas C. Meisel and Ulrike Pitha and Thomas Prohaska and Markus Puschenreiter and Elmar Rückert and Bernhard Spangl and Dominik Wiedenhofer and Johanna Irrgeher},
url = {https://cloud.cps.unileoben.ac.at/index.php/s/WJwtk2JC4XnyzL6},
isbn = {0048-9697},
year = {2024},
date = {2024-05-21},
journal = {Science of The Total Environment},
volume = {934},
note = {https://doi.org/10.1016/j.scitotenv.2024.173364},
keywords = {Applied Deep Learning, environmental health risks, pollution},
pubstate = {published},
tppubtype = {article}
}
| 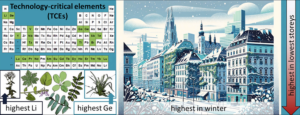 |
Lygerakis, Fotios; Dave, Vedant; Rueckert, Elmar M2CURL: Sample-Efficient Multimodal Reinforcement Learning via Self-Supervised Representation Learning for Robotic Manipulation Proceedings Article In: IEEE International Conference on Ubiquitous Robots (UR 2024), IEEE 2024. @inproceedings{Lygerakis2024,
title = {M2CURL: Sample-Efficient Multimodal Reinforcement Learning via Self-Supervised Representation Learning for Robotic Manipulation},
author = {Fotios Lygerakis and Vedant Dave and Elmar Rueckert},
url = {https://cloud.cps.unileoben.ac.at/index.php/s/NPejb2Fp4Y8LeyZ},
year = {2024},
date = {2024-04-04},
urldate = {2024-04-04},
booktitle = {IEEE International Conference on Ubiquitous Robots (UR 2024)},
organization = {IEEE},
keywords = {Contrastive Learning, Manipulation, Multimodal Reinforcement Learning, Multimodal Representation Learning, Reinforcement Learning, Robot Learning},
pubstate = {published},
tppubtype = {inproceedings}
}
| 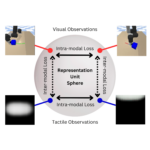 |
Feith, Nikolaus; Rueckert, Elmar Integrating Human Expertise in Continuous Spaces: A Novel Interactive Bayesian Optimization Framework with Preference Expected Improvement Proceedings Article In: IEEE International Conference on Ubiquitous Robots (UR 2024), IEEE 2024. @inproceedings{Feith2024A,
title = {Integrating Human Expertise in Continuous Spaces: A Novel Interactive Bayesian Optimization Framework with Preference Expected Improvement},
author = {Nikolaus Feith and Elmar Rueckert},
url = {https://cloud.cps.unileoben.ac.at/index.php/s/6rTWAkoXa3zsJxf},
year = {2024},
date = {2024-04-04},
urldate = {2024-04-04},
booktitle = {IEEE International Conference on Ubiquitous Robots (UR 2024)},
organization = {IEEE},
keywords = {Interactive Learning, Reinforcement Learning},
pubstate = {published},
tppubtype = {inproceedings}
}
| 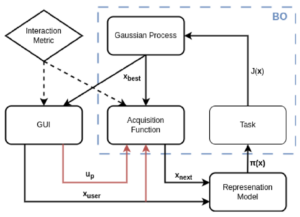 |
Feith, Nikolaus; Rueckert, Elmar Advancing Interactive Robot Learning: A User Interface Leveraging Mixed Reality and Dual Quaternions Proceedings Article In: IEEE International Conference on Ubiquitous Robots (UR 2024), IEEE 2024. @inproceedings{Feith2024B,
title = {Advancing Interactive Robot Learning: A User Interface Leveraging Mixed Reality and Dual Quaternions},
author = {Nikolaus Feith and Elmar Rueckert},
url = {https://cloud.cps.unileoben.ac.at/index.php/s/qCgzbmCJYSH3F97},
year = {2024},
date = {2024-04-04},
urldate = {2024-04-04},
booktitle = {IEEE International Conference on Ubiquitous Robots (UR 2024)},
organization = {IEEE},
keywords = {Human-Robot Interaction, Mixted Reality, Robot Learning},
pubstate = {published},
tppubtype = {inproceedings}
}
| 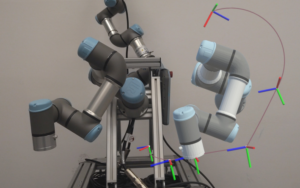 |
Neubauer, Melanie; Rueckert, Elmar Semi-Autonomous Fast Object Segmentation and Tracking Tool for Industrial Applications Proceedings Article In: IEEE International Conference on Ubiquitous Robots (UR 2024), IEEE 2024. @inproceedings{neubauer2024fost,
title = {Semi-Autonomous Fast Object Segmentation and Tracking Tool for Industrial Applications},
author = {Melanie Neubauer and Elmar Rueckert},
url = {https://cloud.cps.unileoben.ac.at/index.php/s/6fTmgDZRQfDHfmg},
year = {2024},
date = {2024-04-04},
urldate = {2024-04-04},
booktitle = {IEEE International Conference on Ubiquitous Robots (UR 2024)},
organization = {IEEE},
keywords = {computer vision, Deep Learning, Recycling},
pubstate = {published},
tppubtype = {inproceedings}
}
| 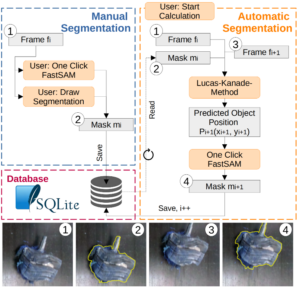 |
Nwankwo, Linus; Rueckert, Elmar Multimodal Human-Autonomous Agents Interaction Using Pre-Trained Language and Visual Foundation Models Workshop 2024, ( In Workshop of the 2024 ACM/IEEE International Conference on HumanRobot Interaction (HRI ’24 Workshop), March 11–14, 2024, Boulder, CO, USA.
ACM, New York, NY, USA). @workshop{Nwankwo2024MultimodalHA,
title = {Multimodal Human-Autonomous Agents Interaction Using Pre-Trained Language and Visual Foundation Models},
author = {Linus Nwankwo and Elmar Rueckert},
url = {https://human-llm-interaction.github.io/workshop/hri24/papers/hllmi24_paper_5.pdf},
year = {2024},
date = {2024-03-11},
urldate = {2024-03-11},
abstract = {In this paper, we extended the method proposed in [17] to enable humans to interact naturally with autonomous agents through vocal and textual conversations. Our extended method exploits the inherent capabilities of pre-trained large language models (LLMs), multimodal visual language models (VLMs), and speech recognition (SR) models to decode the high-level natural language conversations and semantic understanding of the robot's task environment, and abstract them to the robot's actionable commands or queries. We performed a quantitative evaluation of our framework's natural vocal conversation understanding with participants from different racial backgrounds and English language accents. The participants interacted with the robot using both vocal and textual instructional commands. Based on the logged interaction data, our framework achieved 87.55% vocal commands decoding accuracy, 86.27% commands execution success, and an average latency of 0.89 seconds from receiving the participants' vocal chat commands to initiating the robot’s actual physical action. The video demonstrations of this paper can be found at https://linusnep.github.io/MTCC-IRoNL/},
note = { In Workshop of the 2024 ACM/IEEE International Conference on HumanRobot Interaction (HRI ’24 Workshop), March 11–14, 2024, Boulder, CO, USA.
ACM, New York, NY, USA},
keywords = {Autonomous Navigation, Human-Robot Interaction, Large Language Models, mobile navigation},
pubstate = {published},
tppubtype = {workshop}
}
In this paper, we extended the method proposed in [17] to enable humans to interact naturally with autonomous agents through vocal and textual conversations. Our extended method exploits the inherent capabilities of pre-trained large language models (LLMs), multimodal visual language models (VLMs), and speech recognition (SR) models to decode the high-level natural language conversations and semantic understanding of the robot's task environment, and abstract them to the robot's actionable commands or queries. We performed a quantitative evaluation of our framework's natural vocal conversation understanding with participants from different racial backgrounds and English language accents. The participants interacted with the robot using both vocal and textual instructional commands. Based on the logged interaction data, our framework achieved 87.55% vocal commands decoding accuracy, 86.27% commands execution success, and an average latency of 0.89 seconds from receiving the participants' vocal chat commands to initiating the robot’s actual physical action. The video demonstrations of this paper can be found at https://linusnep.github.io/MTCC-IRoNL/ | 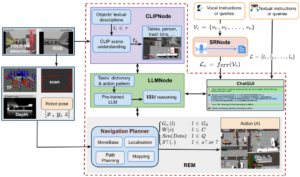 |
Kunavar, Tjasa; Jamšek, Marko; Avila-Mireles, Edwin Johnatan; Rueckert, Elmar; Peternel, Luka; Babič., Jan The Effects of Different Motor Teaching Strategies on Learning a Complex Motor Task Journal Article In: Sensors (MDPI), vol. 24, no. 4, pp. 1–17, 2024. @article{Kunavar2024,
title = {The Effects of Different Motor Teaching Strategies on Learning a Complex Motor Task},
author = {Tjasa Kunavar and Marko Jamšek and Edwin Johnatan Avila-Mireles and Elmar Rueckert and Luka Peternel and Jan Babič. },
url = {https://cloud.cps.unileoben.ac.at/index.php/s/F8ferD4kD7rsnN2},
doi = {10.3390/s24041231},
year = {2024},
date = {2024-02-15},
urldate = {2024-02-15},
journal = {Sensors (MDPI)},
volume = {24},
number = {4},
pages = {1--17},
keywords = {Human motion analysis},
pubstate = {published},
tppubtype = {article}
}
| 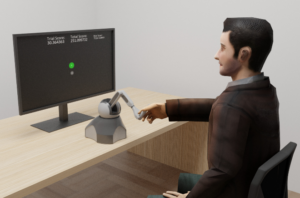 |
Dave*, Vedant; Lygerakis*, Fotios; Rueckert, Elmar Multimodal Visual-Tactile Representation Learning through Self-Supervised Contrastive Pre-Training Proceedings Article In: IEEE International Conference on Robotics and Automation (ICRA), pp. 8013-8020, IEEE, 2024, ISBN: 979-8-3503-8457-4, (* equal contribution). @inproceedings{Dave2024b,
title = {Multimodal Visual-Tactile Representation Learning through Self-Supervised Contrastive Pre-Training},
author = {Vedant Dave* and Fotios Lygerakis* and Elmar Rueckert},
url = {https://cloud.cps.unileoben.ac.at/index.php/s/Nw9TprfdDoLgr8e},
doi = {10.1109/ICRA57147.2024.10610228},
isbn = {979-8-3503-8457-4},
year = {2024},
date = {2024-01-28},
urldate = {2024-01-28},
booktitle = {IEEE International Conference on Robotics and Automation (ICRA)},
pages = {8013-8020},
publisher = {IEEE},
abstract = {The rapidly evolving field of robotics necessitates methods that can facilitate the fusion of multiple modalities. Specifically, when it comes to interacting with tangible objects, effectively combining visual and tactile sensory data is key to understanding and navigating the complex dynamics of the physical world, enabling a more nuanced and adaptable response to changing environments. Nevertheless, much of the earlier work in merging these two sensory modalities has relied on supervised methods utilizing datasets labeled by humans. This paper introduces MViTac, a novel methodology that leverages contrastive learning to integrate vision and touch sensations in a self-supervised fashion. By availing both sensory inputs, MViTac leverages intra and inter-modality losses for learning representations, resulting in enhanced material property classification and more adept grasping prediction. Through a series of experiments, we showcase the effectiveness of our method and its superiority over existing state-of-the-art self-supervised and supervised techniques. In evaluating our methodology, we focus on two distinct tasks: material classification and grasping success prediction. Our results indicate that MViTac facilitates the development of improved modality encoders, yielding more robust representations as evidenced by linear probing assessments. https://sites.google.com/view/mvitac/home},
note = {* equal contribution},
keywords = {Contrastive Learning, Representation Learning, Self-supervised Learning, Tactile Sensing},
pubstate = {published},
tppubtype = {inproceedings}
}
The rapidly evolving field of robotics necessitates methods that can facilitate the fusion of multiple modalities. Specifically, when it comes to interacting with tangible objects, effectively combining visual and tactile sensory data is key to understanding and navigating the complex dynamics of the physical world, enabling a more nuanced and adaptable response to changing environments. Nevertheless, much of the earlier work in merging these two sensory modalities has relied on supervised methods utilizing datasets labeled by humans. This paper introduces MViTac, a novel methodology that leverages contrastive learning to integrate vision and touch sensations in a self-supervised fashion. By availing both sensory inputs, MViTac leverages intra and inter-modality losses for learning representations, resulting in enhanced material property classification and more adept grasping prediction. Through a series of experiments, we showcase the effectiveness of our method and its superiority over existing state-of-the-art self-supervised and supervised techniques. In evaluating our methodology, we focus on two distinct tasks: material classification and grasping success prediction. Our results indicate that MViTac facilitates the development of improved modality encoders, yielding more robust representations as evidenced by linear probing assessments. https://sites.google.com/view/mvitac/home | 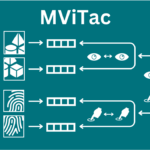 |
Nwankwo, Linus; Rueckert, Elmar The Conversation is the Command: Interacting with Real-World Autonomous Robots Through Natural Language Proceedings Article In: HRI '24: Companion of the 2024 ACM/IEEE International Conference on Human-Robot Interaction., pp. 808–812, ACM/IEEE Association for Computing Machinery, New York, NY, USA, 2024, ISBN: 9798400703232, (Published as late breaking results. Supplementary video: https://cloud.cps.unileoben.ac.at/index.php/s/fRE9XMosWDtJ339 ). @inproceedings{Nwankwo2024,
title = {The Conversation is the Command: Interacting with Real-World Autonomous Robots Through Natural Language},
author = {Linus Nwankwo and Elmar Rueckert},
url = {https://doi.org/10.1145/3610978.3640723
https://cloud.cps.unileoben.ac.at/index.php/s/YzJdHWDt9ZdqsZs},
doi = {10.1145/3610978.3640723},
isbn = {9798400703232},
year = {2024},
date = {2024-01-16},
urldate = {2024-01-16},
booktitle = {HRI '24: Companion of the 2024 ACM/IEEE International Conference on Human-Robot Interaction.},
pages = {808–812},
publisher = {Association for Computing Machinery},
address = {New York, NY, USA},
organization = {ACM/IEEE},
series = {HRI '24},
abstract = {In recent years, autonomous agents have surged in real-world environments such as our homes, offices, and public spaces. However, natural human-robot interaction remains a key challenge. In this paper, we introduce an approach that synergistically exploits the capabilities of large language models (LLMs) and multimodal vision-language models (VLMs) to enable humans to interact naturally with autonomous robots through conversational dialogue. We leveraged the LLMs to decode the high-level natural language instructions from humans and abstract them into precise robot actionable commands or queries. Further, we utilised the VLMs to provide a visual and semantic understanding of the robot's task environment. Our results with 99.13% command recognition accuracy and 97.96% commands execution success show that our approach can enhance human-robot interaction in real-world applications. The video demonstrations of this paper can be found at https://osf.io/wzyf6 and the code is available at our GitHub repository.},
howpublished = {ACM/IEEE International Conference on Human-Robot Interaction (HRI ’24 Companion)},
key = {ChatGPT, LLMs, ROS, VLMs, autonomous robots, human-robot interaction, natural language interaction},
note = {Published as late breaking results. Supplementary video: https://cloud.cps.unileoben.ac.at/index.php/s/fRE9XMosWDtJ339 },
keywords = {Autonomous Navigation, Large Language Models},
pubstate = {published},
tppubtype = {inproceedings}
}
In recent years, autonomous agents have surged in real-world environments such as our homes, offices, and public spaces. However, natural human-robot interaction remains a key challenge. In this paper, we introduce an approach that synergistically exploits the capabilities of large language models (LLMs) and multimodal vision-language models (VLMs) to enable humans to interact naturally with autonomous robots through conversational dialogue. We leveraged the LLMs to decode the high-level natural language instructions from humans and abstract them into precise robot actionable commands or queries. Further, we utilised the VLMs to provide a visual and semantic understanding of the robot's task environment. Our results with 99.13% command recognition accuracy and 97.96% commands execution success show that our approach can enhance human-robot interaction in real-world applications. The video demonstrations of this paper can be found at https://osf.io/wzyf6 and the code is available at our GitHub repository. | 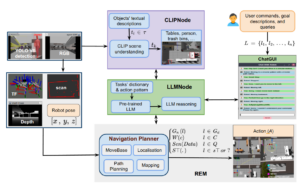 |
2023
|
Lygerakis, Fotios; Rueckert, Elmar CR-VAE: Contrastive Regularization on Variational Autoencoders for Preventing Posterior Collapse Proceedings Article In: Asian Conference of Artificial Intelligence Technology (ACAIT)., IEEE, 2023. @inproceedings{Lygerakis2023,
title = {CR-VAE: Contrastive Regularization on Variational Autoencoders for Preventing Posterior Collapse},
author = {Fotios Lygerakis and Elmar Rueckert},
url = {https://cloud.cps.unileoben.ac.at/index.php/s/fNNRnzJFPrtGQM2},
year = {2023},
date = {2023-08-16},
urldate = {2023-08-16},
booktitle = {Asian Conference of Artificial Intelligence Technology (ACAIT).},
publisher = {IEEE},
keywords = {Representation Learning, Self-supervised Learning},
pubstate = {published},
tppubtype = {inproceedings}
}
| 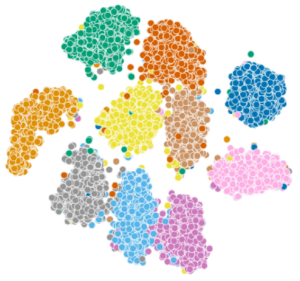 |
Yadav, Harsh; Xue, Honghu; Rudall, Yan; Bakr, Mohamed; Hein, Benedikt; Rueckert, Elmar; Nguyen, Ngoc Thinh Deep Reinforcement Learning for Mapless Navigation of Autonomous Mobile Robot Proceedings Article In: International Conference on System Theory, Control and Computing (ICSTCC), 2023, (October 11-13, 2023, Timisoara, Romania.). @inproceedings{Yadav2023b,
title = {Deep Reinforcement Learning for Mapless Navigation of Autonomous Mobile Robot},
author = {Harsh Yadav and Honghu Xue and Yan Rudall and Mohamed Bakr and Benedikt Hein and Elmar Rueckert and Ngoc Thinh Nguyen},
url = {https://cloud.cps.unileoben.ac.at/index.php/s/zEnY3yoFHZRdzkR},
year = {2023},
date = {2023-06-26},
urldate = {2023-06-26},
publisher = { International Conference on System Theory, Control and Computing (ICSTCC)},
note = {October 11-13, 2023, Timisoara, Romania.},
keywords = {Autonomous Navigation, Deep Learning, Reinforcement Learning},
pubstate = {published},
tppubtype = {inproceedings}
}
| 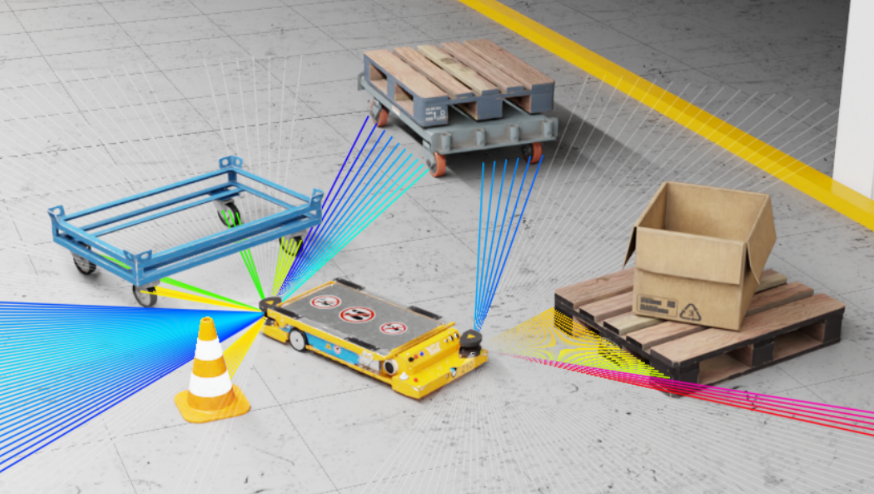 |
Nwankwo, Linus; Rueckert, Elmar Understanding why SLAM algorithms fail in modern indoor environments Proceedings Article In: International Conference on Robotics in Alpe-Adria-Danube Region (RAAD). , pp. 186 - 194, Cham: Springer Nature Switzerland., 2023. @inproceedings{Nwankwo2023,
title = {Understanding why SLAM algorithms fail in modern indoor environments},
author = {Linus Nwankwo and Elmar Rueckert},
url = {https://cloud.cps.unileoben.ac.at/index.php/s/KdZ2E2np5QEnYfL
},
doi = {https://doi.org/10.1007/978-3-031-32606-6_22},
year = {2023},
date = {2023-05-27},
urldate = {2023-05-27},
booktitle = {International Conference on Robotics in Alpe-Adria-Danube Region (RAAD). },
volume = {135},
pages = {186 - 194},
publisher = {Cham: Springer Nature Switzerland.},
series = {Mechanisms and Machine Science},
abstract = {Simultaneous localization and mapping (SLAM) algorithms are essential for the autonomous navigation of mobile robots. With the increasing demand for autonomous systems, it is crucial to evaluate and compare the performance of these algorithms in real-world environments.
In this paper, we provide an evaluation strategy and real-world datasets to test and evaluate SLAM algorithms in complex and challenging indoor environments. Further, we analysed state-of-the-art (SOTA) SLAM algorithms based on various metrics such as absolute trajectory error, scale drift, and map accuracy and consistency. Our results demonstrate that SOTA SLAM algorithms often fail in challenging environments, with dynamic objects, transparent and reflecting surfaces. We also found that successful loop closures had a significant impact on the algorithm’s performance. These findings highlight the need for further research to improve the robustness of the algorithms in real-world scenarios. },
keywords = {mobile navigation, robotics, SLAM},
pubstate = {published},
tppubtype = {inproceedings}
}
Simultaneous localization and mapping (SLAM) algorithms are essential for the autonomous navigation of mobile robots. With the increasing demand for autonomous systems, it is crucial to evaluate and compare the performance of these algorithms in real-world environments.
In this paper, we provide an evaluation strategy and real-world datasets to test and evaluate SLAM algorithms in complex and challenging indoor environments. Further, we analysed state-of-the-art (SOTA) SLAM algorithms based on various metrics such as absolute trajectory error, scale drift, and map accuracy and consistency. Our results demonstrate that SOTA SLAM algorithms often fail in challenging environments, with dynamic objects, transparent and reflecting surfaces. We also found that successful loop closures had a significant impact on the algorithm’s performance. These findings highlight the need for further research to improve the robustness of the algorithms in real-world scenarios. | 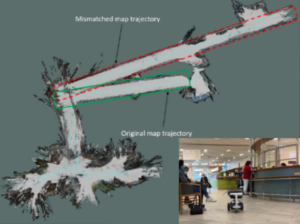 |
Nwankwo, Linus; Fritze, Clemens; Bartsch, Konrad; Rueckert, Elmar ROMR: A ROS-based Open-source Mobile Robot Journal Article In: HardwareX, vol. 15, pp. 1–29, 2023. @article{Nwankwo2023b,
title = {ROMR: A ROS-based Open-source Mobile Robot},
author = {Linus Nwankwo and Clemens Fritze and Konrad Bartsch and Elmar Rueckert},
url = {https://cloud.cps.unileoben.ac.at/index.php/s/8aXLXXPFAZ4wq54},
doi = {10.1016/j.ohx.2023.e00426},
year = {2023},
date = {2023-04-17},
urldate = {2023-04-17},
journal = {HardwareX},
volume = {15},
pages = {1--29},
abstract = {Currently, commercially available intelligent transport robots that are capable of carrying up to 90kg of load can cost $5,000 or even more. This makes real-world experimentation prohibitively expensive, and limiting the applicability of such systems to everyday home or industrial tasks. Aside from their high cost, the majority of commercially available platforms are either closed-source, platform-specific, or use difficult-to-customize hardware and firmware. In this work, we present a low-cost, open-source and modular alternative, referred to herein as ”ROS-based open-source mobile robot (ROMR)”. ROMR utilizes off-the-shelf (OTS) components, additive manufacturing technologies, aluminium profiles, and a consumer hoverboard with high-torque brushless direct current (BLDC) motors. ROMR is fully compatible with the robot operating system (ROS), has a maximum payload of 90kg, and costs less than $1500. Furthermore, ROMR offers a simple yet robust framework for contextualizing simultaneous localization and mapping (SLAM) algorithms, an essential prerequisite for autonomous robot navigation. The robustness and performance of the ROMR were validated through realworld and simulation experiments. All the design, construction and software files are freely available online under the GNU GPL v3 license at https://doi.org/10.17605/OSF.IO/K83X7. A descriptive video of ROMR can be found at https://osf.io/ku8ag.},
keywords = {Autonomous Navigation, mobile navigation, SLAM},
pubstate = {published},
tppubtype = {article}
}
Currently, commercially available intelligent transport robots that are capable of carrying up to 90kg of load can cost $5,000 or even more. This makes real-world experimentation prohibitively expensive, and limiting the applicability of such systems to everyday home or industrial tasks. Aside from their high cost, the majority of commercially available platforms are either closed-source, platform-specific, or use difficult-to-customize hardware and firmware. In this work, we present a low-cost, open-source and modular alternative, referred to herein as ”ROS-based open-source mobile robot (ROMR)”. ROMR utilizes off-the-shelf (OTS) components, additive manufacturing technologies, aluminium profiles, and a consumer hoverboard with high-torque brushless direct current (BLDC) motors. ROMR is fully compatible with the robot operating system (ROS), has a maximum payload of 90kg, and costs less than $1500. Furthermore, ROMR offers a simple yet robust framework for contextualizing simultaneous localization and mapping (SLAM) algorithms, an essential prerequisite for autonomous robot navigation. The robustness and performance of the ROMR were validated through realworld and simulation experiments. All the design, construction and software files are freely available online under the GNU GPL v3 license at https://doi.org/10.17605/OSF.IO/K83X7. A descriptive video of ROMR can be found at https://osf.io/ku8ag. | 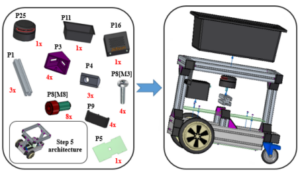 |
Yadav, Harsh; Xue, Honghu; Rudall, Yan; Bakr, Mohamed; Hein, Benedikt; Rueckert, Elmar; Nguyen, Thinh Deep Reinforcement Learning for Autonomous Navigation in Intralogistics Workshop 2023, (European Control Conference (ECC) Workshop, Extended Abstract.). @workshop{Yadav2023,
title = {Deep Reinforcement Learning for Autonomous Navigation in Intralogistics},
author = {Harsh Yadav and Honghu Xue and Yan Rudall and Mohamed Bakr and Benedikt Hein and Elmar Rueckert and Thinh Nguyen},
url = {https://cloud.cps.unileoben.ac.at/index.php/s/tw4D43WTzG6yLmE},
year = {2023},
date = {2023-03-10},
urldate = {2023-03-10},
abstract = {Even with several advances in autonomous mobile robots, navigation in a highly dynamic environment still remains a challenge. Classical navigation systems, such as Simultaneous Localization and Mapping (SLAM), build a map of the environment and constructing maps of highly dynamic environments is impractical. Deep Reinforcement Learning (DRL) approaches have the ability to learn policies without knowledge of the maps or the transition models of the environment.
The aim of our work is to investigate the potential of using DRL to control an autonomous mobile robot to dock with a load carrier. This paper presents an initial successful training result of the Soft Actor-Critic (SAC) algorithm, which can navigate a robot toward an open door only based on the 360° LiDAR observations. Ongoing work is using visual sensors for load carrier docking.},
howpublished = {European Control Conference (ECC)},
note = {European Control Conference (ECC) Workshop, Extended Abstract.},
keywords = {Autonomous Navigation, Deep Learning, mobile navigation, SLAM},
pubstate = {published},
tppubtype = {workshop}
}
Even with several advances in autonomous mobile robots, navigation in a highly dynamic environment still remains a challenge. Classical navigation systems, such as Simultaneous Localization and Mapping (SLAM), build a map of the environment and constructing maps of highly dynamic environments is impractical. Deep Reinforcement Learning (DRL) approaches have the ability to learn policies without knowledge of the maps or the transition models of the environment.
The aim of our work is to investigate the potential of using DRL to control an autonomous mobile robot to dock with a load carrier. This paper presents an initial successful training result of the Soft Actor-Critic (SAC) algorithm, which can navigate a robot toward an open door only based on the 360° LiDAR observations. Ongoing work is using visual sensors for load carrier docking. | 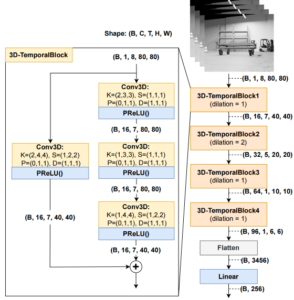 |
Keshavarz, Sahar; Vita, Petr; Rueckert, Elmar; Ortner, Ronald; Thonhauser, Gerhard A Reinforcement Learning Approach for Real-Time Autonomous Decision-Making in Well Construction Proceedings Article In: Society of Petroleum Engineers - SPE Symposium: Leveraging Artificial Intelligence to Shape the Future of the Energy Industry, AIS 2023, Society of Petroleum Engineers., 2023, ISBN: 9781613999882. @inproceedings{Keshavarz2023,
title = {A Reinforcement Learning Approach for Real-Time Autonomous Decision-Making in Well Construction},
author = {Sahar Keshavarz and Petr Vita and Elmar Rueckert and Ronald Ortner and Gerhard Thonhauser},
url = {https://cloud.cps.unileoben.ac.at/index.php/s/yT9Erwsnk36JKtr},
doi = {10.2118/214465-MS},
isbn = {9781613999882},
year = {2023},
date = {2023-01-19},
urldate = {2023-01-19},
booktitle = {Society of Petroleum Engineers - SPE Symposium: Leveraging Artificial Intelligence to Shape the Future of the Energy Industry, AIS 2023},
publisher = {Society of Petroleum Engineers.},
keywords = {Reinforcement Learning, Well Construction},
pubstate = {published},
tppubtype = {inproceedings}
}
| 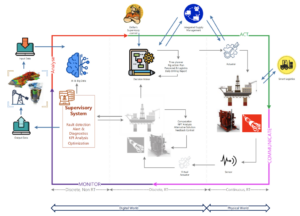 |
2022
|
Dave, Vedant; Rueckert, Elmar Can we infer the full-arm manipulation skills from tactile targets? Workshop Advances in Close Proximity Human-Robot Collaboration Workshop, International Conference on Humanoid Robots (Humanoids), 2022. @workshop{Dave2022WS,
title = {Can we infer the full-arm manipulation skills from tactile targets?},
author = {Vedant Dave and Elmar Rueckert},
url = {https://cloud.cps.unileoben.ac.at/index.php/f/562953},
year = {2022},
date = {2022-11-28},
urldate = {2022-11-28},
booktitle = {Advances in Close Proximity Human-Robot Collaboration Workshop},
publisher = {International Conference on Humanoid Robots (Humanoids)},
abstract = {Tactile sensing provides significant information about the state of the environment for performing manipulation tasks. Manipulation skills depends on the desired initial contact points between the object and the end-effector. Based on physical properties of the object, this contact results into distinct tactile responses. We propose Tactile Probabilistic Movement Primitives (TacProMPs), to learn a highly non-linear relationship between the desired tactile responses and the full-arm movement, where we condition solely on the tactile responses to infer the complex manipulation skills. We use a Gaussian mixture model of primitives to address the multimodality in demonstrations. We demonstrate the performance of our method in challenging real-world scenarios.},
keywords = {Grasping, Manipulation, Probabilistic Movement Primitives, Tactile Sensing},
pubstate = {published},
tppubtype = {workshop}
}
Tactile sensing provides significant information about the state of the environment for performing manipulation tasks. Manipulation skills depends on the desired initial contact points between the object and the end-effector. Based on physical properties of the object, this contact results into distinct tactile responses. We propose Tactile Probabilistic Movement Primitives (TacProMPs), to learn a highly non-linear relationship between the desired tactile responses and the full-arm movement, where we condition solely on the tactile responses to infer the complex manipulation skills. We use a Gaussian mixture model of primitives to address the multimodality in demonstrations. We demonstrate the performance of our method in challenging real-world scenarios. | 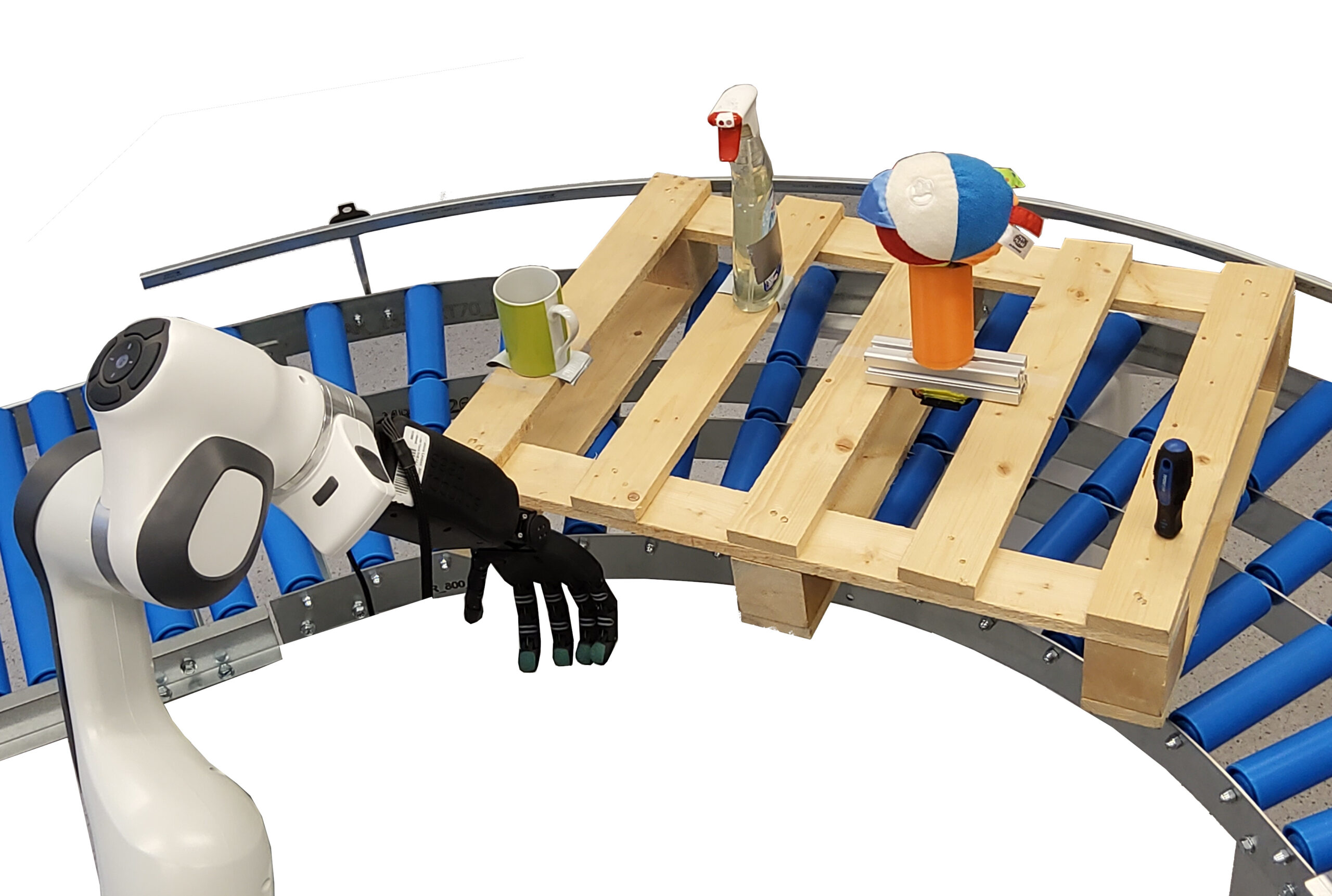 |
Xue, Honghu; Song, Rui; Petzold, Julian; Hein, Benedikt; Hamann, Heiko; Rueckert, Elmar End-To-End Deep Reinforcement Learning for First-Person Pedestrian Visual Navigation in Urban Environments Proceedings Article In: International Conference on Humanoid Robots (Humanoids 2022), 2022. @inproceedings{Xue2022b,
title = {End-To-End Deep Reinforcement Learning for First-Person Pedestrian Visual Navigation in Urban Environments},
author = {Honghu Xue and Rui Song and Julian Petzold and Benedikt Hein and Heiko Hamann and Elmar Rueckert},
url = {https://cloud.cps.unileoben.ac.at/index.php/s/RzMQWqsFarQ6Kw4},
year = {2022},
date = {2022-09-26},
urldate = {2022-09-26},
publisher = {International Conference on Humanoid Robots (Humanoids 2022)},
abstract = {We solve a visual navigation problem in an urban setting via deep reinforcement learning in an end-to-end manner. A major challenge of a first-person visual navigation problem lies in severe partial observability and sparse positive experiences of reaching the goal. To address partial observability, we propose a novel 3D-temporal convolutional network to encode sequential historical visual observations, its effectiveness is verified by comparing to a commonly-used frame-stacking
approach. For sparse positive samples, we propose an improved automatic curriculum learning algorithm NavACL+, which
proposes meaningful curricula starting from easy tasks and gradually generalizes to challenging ones. NavACL+ is shown to
facilitate the learning process, greatly improve the task success rate on difficult tasks by at least 40% and offer enhanced
generalization to different initial poses compared to training from a fixed initial pose and the original NavACL algorithm.},
keywords = {Autonomous Navigation, Deep Learning, mobile navigation},
pubstate = {published},
tppubtype = {inproceedings}
}
We solve a visual navigation problem in an urban setting via deep reinforcement learning in an end-to-end manner. A major challenge of a first-person visual navigation problem lies in severe partial observability and sparse positive experiences of reaching the goal. To address partial observability, we propose a novel 3D-temporal convolutional network to encode sequential historical visual observations, its effectiveness is verified by comparing to a commonly-used frame-stacking
approach. For sparse positive samples, we propose an improved automatic curriculum learning algorithm NavACL+, which
proposes meaningful curricula starting from easy tasks and gradually generalizes to challenging ones. NavACL+ is shown to
facilitate the learning process, greatly improve the task success rate on difficult tasks by at least 40% and offer enhanced
generalization to different initial poses compared to training from a fixed initial pose and the original NavACL algorithm. | 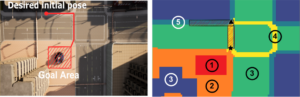 |
Dave, Vedant; Rueckert, Elmar Predicting full-arm grasping motions from anticipated tactile responses Proceedings Article In: International Conference on Humanoid Robots (Humanoids), pp. 464-471, IEEE, 2022, ISBN: 979-8-3503-0979-9. @inproceedings{Dave2022,
title = {Predicting full-arm grasping motions from anticipated tactile responses},
author = {Vedant Dave and Elmar Rueckert},
url = {https://cloud.cps.unileoben.ac.at/index.php/s/WzGSNtc5WRLN3EL},
doi = {10.1109/Humanoids53995.2022.9999743},
isbn = {979-8-3503-0979-9},
year = {2022},
date = {2022-09-26},
urldate = {2022-09-26},
booktitle = {International Conference on Humanoid Robots (Humanoids)},
pages = {464-471},
publisher = {IEEE},
abstract = {Tactile sensing provides significant information about the state of the environment for performing manipulation tasks. Depending on the physical properties of the object, manipulation tasks can exhibit large variation in their movements. For a grasping task, the movement of the arm and of the end effector varies depending on different points of contact on the object, especially if the object is non-homogeneous in hardness and/or has an uneven geometry. In this paper, we propose Tactile Probabilistic Movement Primitives (TacProMPs), to learn a highly non-linear relationship between the desired tactile responses and the full-arm movement. We solely condition on the tactile responses to infer the complex manipulation skills. We formulate a joint trajectory of full-arm joints with tactile data, leverage the model to condition on the desired tactile response from the non-homogeneous object and infer the full-arm (7-dof panda arm and 19-dof gripper hand) motion. We use a Gaussian Mixture Model of primitives to address the multimodality in demonstrations. We also show that the measurement noise adjustment must be taken into account due to multiple systems working in collaboration.
We validate and show the robustness of the approach through two experiments. First, we consider an object with non-uniform hardness. Grasping from different locations require different motion, and results into different tactile responses. Second, we have an object with homogeneous hardness, but we grasp it with widely varying grasping configurations. Our result shows that TacProMPs can successfully model complex multimodal skills and generalise to new situations.},
keywords = {Grasping, Manipulation, Probabilistic Movement Primitives, Tactile Sensing},
pubstate = {published},
tppubtype = {inproceedings}
}
Tactile sensing provides significant information about the state of the environment for performing manipulation tasks. Depending on the physical properties of the object, manipulation tasks can exhibit large variation in their movements. For a grasping task, the movement of the arm and of the end effector varies depending on different points of contact on the object, especially if the object is non-homogeneous in hardness and/or has an uneven geometry. In this paper, we propose Tactile Probabilistic Movement Primitives (TacProMPs), to learn a highly non-linear relationship between the desired tactile responses and the full-arm movement. We solely condition on the tactile responses to infer the complex manipulation skills. We formulate a joint trajectory of full-arm joints with tactile data, leverage the model to condition on the desired tactile response from the non-homogeneous object and infer the full-arm (7-dof panda arm and 19-dof gripper hand) motion. We use a Gaussian Mixture Model of primitives to address the multimodality in demonstrations. We also show that the measurement noise adjustment must be taken into account due to multiple systems working in collaboration.
We validate and show the robustness of the approach through two experiments. First, we consider an object with non-uniform hardness. Grasping from different locations require different motion, and results into different tactile responses. Second, we have an object with homogeneous hardness, but we grasp it with widely varying grasping configurations. Our result shows that TacProMPs can successfully model complex multimodal skills and generalise to new situations. | 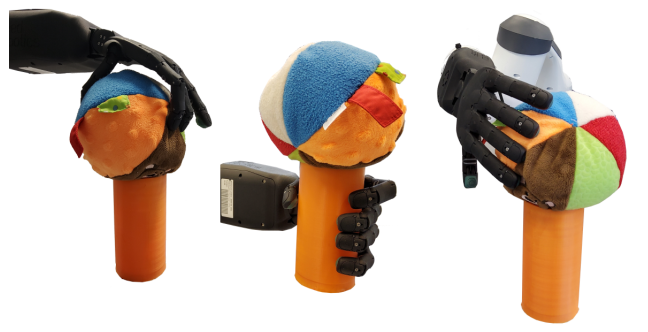 |
Herzog, Rebecca; Berger, Till M; Pauly, Martje Gesine; Xue, Honghu; Rueckert, Elmar; Munchau, Alexander; B"aumer, Tobias; Weissbach, Anne Cerebellar transcranial current stimulation-an intraindividual comparison of different techniques Journal Article In: Frontiers in Neuroscience, 2022. @article{Herzog2022,
title = {Cerebellar transcranial current stimulation-an intraindividual comparison of different techniques},
author = {Rebecca Herzog and Till M Berger and Martje Gesine Pauly and Honghu Xue and Elmar Rueckert and Alexander Munchau and Tobias B{"a}umer and Anne Weissbach},
url = {https://cloud.cps.unileoben.ac.at/index.php/s/4qoooTyjFfBwYEZ},
doi = {10.3389/fnins.2022.987472},
year = {2022},
date = {2022-09-19},
urldate = {2022-09-19},
journal = {Frontiers in Neuroscience},
keywords = {Human motion analysis, human motor control, machine learning, Medical Robotics},
pubstate = {published},
tppubtype = {article}
}
| 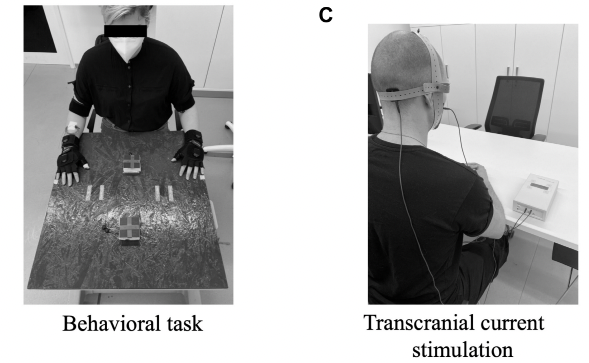 |
Rottmann, Nils; Studt, Nico; Ernst, Floris; Rueckert, Elmar ROS-Mobile: An Android™ application for the Robot Operating System Journal Article In: Arxiv, 2022. @article{Rottmann2022,
title = {ROS-Mobile: An Android™ application for the Robot Operating System},
author = {Nils Rottmann and Nico Studt and Floris Ernst and Elmar Rueckert},
url = {https://arxiv.org/pdf/2011.02781.pdf},
doi = {10.48550/arXiv.2011.02781},
year = {2022},
date = {2022-09-01},
urldate = {2022-09-01},
journal = {Arxiv},
keywords = {Autonomous Navigation, mobile navigation, Simulation},
pubstate = {published},
tppubtype = {article}
}
| 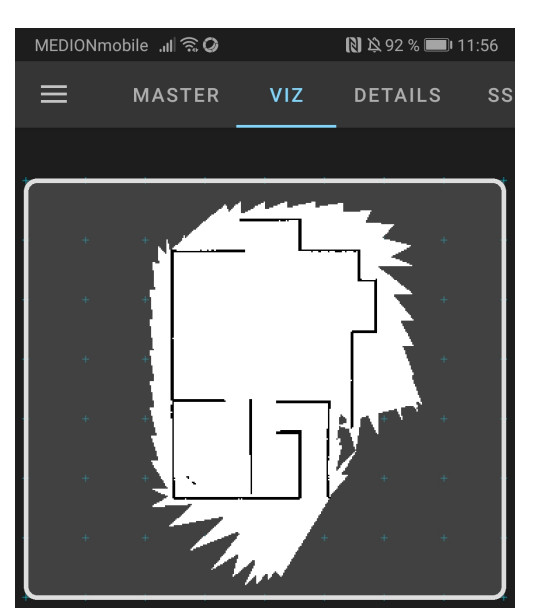 |
Xue, Honghu; Hein, Benedikt; Bakr, Mohamed; Schildbach, Georg; Abel, Bengt; Rueckert, Elmar Using Deep Reinforcement Learning with Automatic Curriculum Learning for Mapless Navigation in Intralogistics Journal Article In: Applied Sciences (MDPI), Special Issue on Intelligent Robotics, 2022, (Supplement: https://cloud.cps.unileoben.ac.at/index.php/s/Sj68rQewnkf4ppZ). @article{Xue2022,
title = {Using Deep Reinforcement Learning with Automatic Curriculum Learning for Mapless Navigation in Intralogistics},
author = {Honghu Xue and Benedikt Hein and Mohamed Bakr and Georg Schildbach and Bengt Abel and Elmar Rueckert},
editor = {/},
url = {https://cloud.cps.unileoben.ac.at/index.php/s/yddDZ7z9oqxenCi
},
year = {2022},
date = {2022-01-31},
urldate = {2022-01-31},
journal = {Applied Sciences (MDPI), Special Issue on Intelligent Robotics},
abstract = {We propose a deep reinforcement learning approach for solving a mapless navigation problem in warehouse scenarios. The automatic guided vehicle is equipped with LiDAR and frontal RGB sensors and learns to reach underneath the target dolly. The challenges reside in the sparseness of positive samples for learning, multi-modal sensor perception with partial observability, the demand for accurate steering maneuvers together with long training cycles. To address these points, we proposed NavACL-Q as an automatic curriculum learning together with distributed soft actor-critic. The performance of the learning algorithm is evaluated exhaustively in a different warehouse environment to check both robustness and generalizability of the learned policy. Results in NVIDIA Isaac Sim demonstrates that our trained agent significantly outperforms the map-based navigation pipeline provided by NVIDIA Isaac Sim in terms of higher agent-goal distances and relative orientations. The ablation studies also confirmed that NavACL-Q greatly facilitates the whole learning process and a pre-trained feature extractor manifestly boosts the training speed.},
note = {Supplement: https://cloud.cps.unileoben.ac.at/index.php/s/Sj68rQewnkf4ppZ},
keywords = {Autonomous Navigation, Deep Learning, mobile navigation, Reinforcement Learning},
pubstate = {published},
tppubtype = {article}
}
We propose a deep reinforcement learning approach for solving a mapless navigation problem in warehouse scenarios. The automatic guided vehicle is equipped with LiDAR and frontal RGB sensors and learns to reach underneath the target dolly. The challenges reside in the sparseness of positive samples for learning, multi-modal sensor perception with partial observability, the demand for accurate steering maneuvers together with long training cycles. To address these points, we proposed NavACL-Q as an automatic curriculum learning together with distributed soft actor-critic. The performance of the learning algorithm is evaluated exhaustively in a different warehouse environment to check both robustness and generalizability of the learned policy. Results in NVIDIA Isaac Sim demonstrates that our trained agent significantly outperforms the map-based navigation pipeline provided by NVIDIA Isaac Sim in terms of higher agent-goal distances and relative orientations. The ablation studies also confirmed that NavACL-Q greatly facilitates the whole learning process and a pre-trained feature extractor manifestly boosts the training speed. | 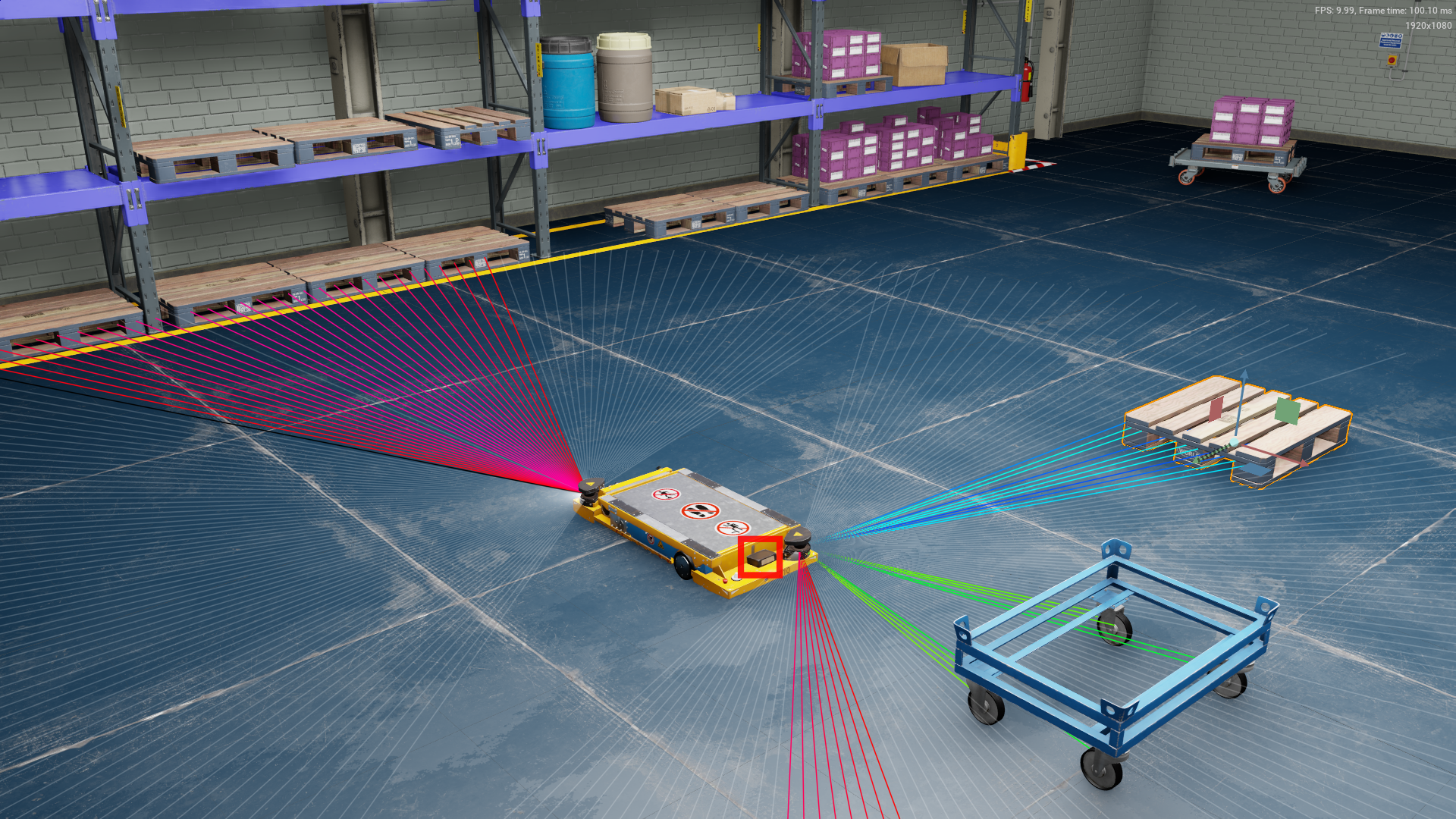 |
Leonel, Rozo*; Vedant, Dave* Orientation Probabilistic Movement Primitives on Riemannian Manifolds Proceedings Article In: Conference on Robot Learning (CoRL), pp. 11, 2022, (* equal contribution). @inproceedings{Leonel2022,
title = {Orientation Probabilistic Movement Primitives on Riemannian Manifolds},
author = {Leonel, Rozo* and Vedant, Dave*},
url = {https://cps.unileoben.ac.at/wp/orientation_probabilistic_move.pdf, Article File},
year = {2022},
date = {2022-01-11},
urldate = {2022-01-11},
booktitle = {Conference on Robot Learning (CoRL)},
volume = {164},
pages = {11},
abstract = {Learning complex robot motions necessarily demands to have models that are able to encode and retrieve full-pose trajectories when tasks are defined in operational spaces. Probabilistic movement primitives (ProMPs) stand out as a principled approach that models trajectory distributions learned from demonstrations. ProMPs allow for trajectory modulation and blending to achieve better generalization to novel situations. However, when ProMPs are employed in operational space, their original formulation does not directly apply to full-pose movements including rotational trajectories described by quaternions. This paper proposes a Riemannian formulation of ProMPs that enables encoding and retrieving of quaternion trajectories. Our method builds on Riemannian manifold theory,
and exploits multilinear geodesic regression for estimating the ProMPs parameters. This novel approach makes ProMPs a suitable model for learning complex full-pose robot motion patterns. Riemannian ProMPs are tested on toy examples to illustrate their workflow, and on real learning-from-demonstration experiments.},
note = {* equal contribution},
keywords = {Manipulation, Probabilistic Movement Primitives, Riemannian Manifolds},
pubstate = {published},
tppubtype = {inproceedings}
}
Learning complex robot motions necessarily demands to have models that are able to encode and retrieve full-pose trajectories when tasks are defined in operational spaces. Probabilistic movement primitives (ProMPs) stand out as a principled approach that models trajectory distributions learned from demonstrations. ProMPs allow for trajectory modulation and blending to achieve better generalization to novel situations. However, when ProMPs are employed in operational space, their original formulation does not directly apply to full-pose movements including rotational trajectories described by quaternions. This paper proposes a Riemannian formulation of ProMPs that enables encoding and retrieving of quaternion trajectories. Our method builds on Riemannian manifold theory,
and exploits multilinear geodesic regression for estimating the ProMPs parameters. This novel approach makes ProMPs a suitable model for learning complex full-pose robot motion patterns. Riemannian ProMPs are tested on toy examples to illustrate their workflow, and on real learning-from-demonstration experiments. | 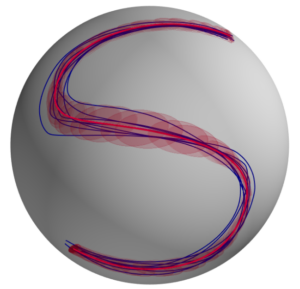 |
2021
|
Denz, R.; Demirci, R.; Cansev, E.; Bliek, A.; Beckerle, P.; Rueckert, E.; Rottmann, N. A high-accuracy, low-budget Sensor Glove for Trajectory Model Learning Proceedings Article In: International Conference on Advanced Robotics , pp. 7, 2021. @inproceedings{Denz2021,
title = {A high-accuracy, low-budget Sensor Glove for Trajectory Model Learning},
author = {R. Denz and R. Demirci and E. Cansev and A. Bliek and P. Beckerle and E. Rueckert and N. Rottmann},
url = {https://cps.unileoben.ac.at/wp/ICAR2021Denz.pdf, Article File},
year = {2021},
date = {2021-12-06},
booktitle = {International Conference on Advanced Robotics },
pages = {7},
keywords = {Grasping, human motor control, Manipulation, smart sensors},
pubstate = {published},
tppubtype = {inproceedings}
}
| 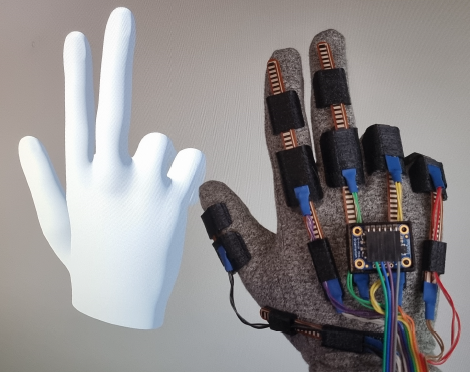 |
Rottmann, N.; Denz, R.; Bruder, R.; Rueckert, E. Probabilistic Approach for Complete Coverage Path Planning with low-cost Systems Proceedings Article In: European Conference on Mobile Robots (ECMR 2021), 2021. @inproceedings{Rottmann2021,
title = {Probabilistic Approach for Complete Coverage Path Planning with low-cost Systems},
author = {N. Rottmann and R. Denz and R. Bruder and E. Rueckert},
url = {https://cps.unileoben.ac.at/wp/ECMR2021Rottmann.pdf, Article File},
year = {2021},
date = {2021-08-31},
booktitle = {European Conference on Mobile Robots (ECMR 2021)},
keywords = {mobile navigation, Probabilistic Inference},
pubstate = {published},
tppubtype = {inproceedings}
}
| 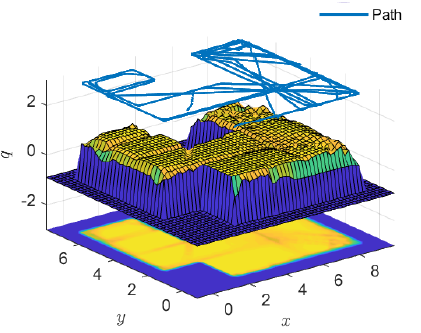 |
Xue, Honghu; Herzog, Rebecca; Berger, Till M.; Bäumer, Tobias; Weissbach, Anne; Rueckert, Elmar Using Probabilistic Movement Primitives in analyzing human motion differences under Transcranial Current Stimulation Journal Article In: Frontiers in Robotics and AI , vol. 8, 2021, ISSN: 2296-9144. @article{Rueckert2021,
title = {Using Probabilistic Movement Primitives in analyzing human motion differences under Transcranial Current Stimulation},
author = {Honghu Xue and Rebecca Herzog and Till M. Berger and Tobias Bäumer and Anne Weissbach and Elmar Rueckert},
editor = {Kensuke Harada},
url = {https://cps.unileoben.ac.at/wp/Frontiers2021Xue.pdf},
issn = {2296-9144},
year = {2021},
date = {2021-08-14},
urldate = {2021-08-14},
journal = {Frontiers in Robotics and AI },
volume = {8},
abstract = {In medical tasks such as human motion analysis, computer-aided auxiliary systems have become preferred choice for human experts for its high efficiency. However, conventional approaches are typically based on user-defined features such as movement onset times, peak velocities, motion vectors or frequency domain analyses. Such approaches entail careful data post-processing or specific domain knowledge to achieve a meaningful feature extraction. Besides, they are prone to noise and the manual-defined features could hardly be re-used for other analyses. In this paper, we proposed probabilistic movement primitives(ProMPs), a widely-used approach in robot skill learning, to model human motions. The benefit of ProMPs is that the features are directly learned from the data and ProMPs can capture important features describing the trajectory shape, which can easily be extended to other tasks. Distinct from previous research, where classification tasks are mostly investigated, we applied ProMPs together with a variant of Kullback-Leibler (KL) divergence to quantify the effect of different transcranial current stimulation methods on human motions. We presented an initial result with10participants. The results validate ProMPs as a robust and effective feature extractor for human motions.},
keywords = {Finger Tapping Motion, Human motion analysis, machine learning, Probabilistic Movement Primitives, Transcranial current stimulation},
pubstate = {published},
tppubtype = {article}
}
In medical tasks such as human motion analysis, computer-aided auxiliary systems have become preferred choice for human experts for its high efficiency. However, conventional approaches are typically based on user-defined features such as movement onset times, peak velocities, motion vectors or frequency domain analyses. Such approaches entail careful data post-processing or specific domain knowledge to achieve a meaningful feature extraction. Besides, they are prone to noise and the manual-defined features could hardly be re-used for other analyses. In this paper, we proposed probabilistic movement primitives(ProMPs), a widely-used approach in robot skill learning, to model human motions. The benefit of ProMPs is that the features are directly learned from the data and ProMPs can capture important features describing the trajectory shape, which can easily be extended to other tasks. Distinct from previous research, where classification tasks are mostly investigated, we applied ProMPs together with a variant of Kullback-Leibler (KL) divergence to quantify the effect of different transcranial current stimulation methods on human motions. We presented an initial result with10participants. The results validate ProMPs as a robust and effective feature extractor for human motions. | 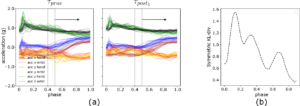 |
Tanneberg, Daniel; Ploeger, Kai; Rueckert, Elmar; Peters, Jan SKID RAW: Skill Discovery from Raw Trajectories Journal Article In: IEEE Robotics and Automation Letters (RA-L), pp. 1–8, 2021, ISSN: 2377-3766, (© 20XX IEEE. Personal use of this material is permitted. Permission from IEEE must be obtained for all other uses, in any current or future media, including reprinting/republishing this material for advertising or promotional purposes, creating new collective works, for resale or redistribution to servers or lists, or reuse of any copyrighted component of this work in other works.). @article{Tanneberg2021,
title = {SKID RAW: Skill Discovery from Raw Trajectories},
author = {Daniel Tanneberg and Kai Ploeger and Elmar Rueckert and Jan Peters },
url = {https://cps.unileoben.ac.at/wp/RAL2021Tanneberg.pdf, Article File},
issn = {2377-3766},
year = {2021},
date = {2021-03-10},
journal = {IEEE Robotics and Automation Letters (RA-L)},
pages = {1--8},
note = {© 20XX IEEE. Personal use of this material is permitted. Permission from IEEE must be obtained for all other uses, in any current or future media, including reprinting/republishing this material for advertising or promotional purposes, creating new collective works, for resale or redistribution to servers or lists, or reuse of any copyrighted component of this work in other works.},
keywords = {Manipulation, movement primitives, Probabilistic Inference},
pubstate = {published},
tppubtype = {article}
}
| 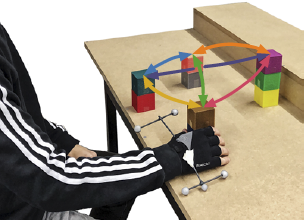 |
Jamsek, Marko; Kunavar, Tjasa; Bobek, Urban; Rueckert, Elmar; Babic, Jan Predictive exoskeleton control for arm-motion augmentation based on probabilistic movement primitives combined with a flow controller Journal Article In: IEEE Robotics and Automation Letters (RA-L), pp. 1–8, 2021, ISSN: 2377-3766, (© 20XX IEEE. Personal use of this material is permitted. Permission from IEEE must be obtained for all other uses, in any current or future media, including reprinting/republishing this material for advertising or promotional purposes, creating new collective works, for resale or redistribution to servers or lists, or reuse of any copyrighted component of this work in other works.). @article{Jamsek2021,
title = {Predictive exoskeleton control for arm-motion augmentation based on probabilistic movement primitives combined with a flow controller},
author = {Marko Jamsek and Tjasa Kunavar and Urban Bobek and Elmar Rueckert and Jan Babic},
url = {../wp/RAL2021Jamsek.pdf, Article File},
doi = {10.1109/LRA.2021.3068892},
issn = {2377-3766},
year = {2021},
date = {2021-03-10},
journal = {IEEE Robotics and Automation Letters (RA-L)},
pages = {1--8},
note = {© 20XX IEEE. Personal use of this material is permitted. Permission from IEEE must be obtained for all other uses, in any current or future media, including reprinting/republishing this material for advertising or promotional purposes, creating new collective works, for resale or redistribution to servers or lists, or reuse of any copyrighted component of this work in other works.},
keywords = {human motor control, movement primitives},
pubstate = {published},
tppubtype = {article}
}
| 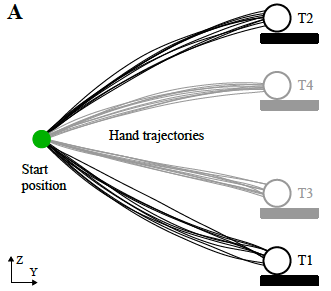 |
Cansev, Mehmet Ege; Xue, Honghu; Rottmann, Nils; Bliek, Adna; Miller, Luke E.; Rueckert, Elmar; Beckerle, Philipp Interactive Human-Robot Skill Transfer: A Review of Learning Methods and User Experience Journal Article In: Advanced Intelligent Systems, pp. 1–28, 2021. @article{Cansev2021,
title = {Interactive Human-Robot Skill Transfer: A Review of Learning Methods and User Experience},
author = {Mehmet Ege Cansev and Honghu Xue and Nils Rottmann and Adna Bliek and Luke E. Miller and Elmar Rueckert and Philipp Beckerle},
url = {https://cps.unileoben.ac.at/wp/AIS2021Cansev.pdf, Article File},
doi = {10.1002/aisy.202000247},
year = {2021},
date = {2021-03-10},
journal = {Advanced Intelligent Systems},
pages = {1--28},
keywords = {human motor control, intrinsic motivation, movement primitives, Probabilistic Inference, Reinforcement Learning, spiking},
pubstate = {published},
tppubtype = {article}
}
| 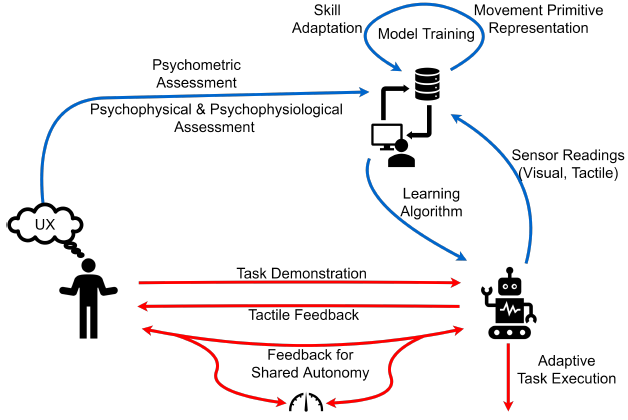 |
2020
|
Akbulut, M Tuluhan; Oztop, Erhan; Seker, M Yunus; Xue, Honghu; Tekden, Ahmet E; Ugur, Emre ACNMP: Skill Transfer and Task Extrapolation through Learning from Demonstration and Reinforcement Learning via Representation Sharing Proceedings Article In: 2020. @inproceedings{nokey,
title = {ACNMP: Skill Transfer and Task Extrapolation through Learning from Demonstration and Reinforcement Learning via Representation Sharing},
author = {M Tuluhan Akbulut and Erhan Oztop and M Yunus Seker and Honghu Xue and Ahmet E Tekden and Emre Ugur},
url = {https://cps.unileoben.ac.at/wp/CoRL2020Akbulut.pdf},
year = {2020},
date = {2020-11-20},
urldate = {2020-11-20},
abstract = {To equip robots with dexterous skills, an effective approach is to first transfer the desired skill via Learning from Demonstration (LfD), then let the robot improve it by self-exploration via Reinforcement Learning (RL). In this paper, we propose a novel LfD+RL framework, namely Adaptive Conditional Neural Movement Primitives (ACNMP), that allows efficient policy improvement in novel environments and effective skill transfer between different agents. This is achieved through exploiting the latent representation learned by the underlying Conditional Neural Process (CNP) model, and simultaneous training of the model with supervised learning (SL) for acquiring the demonstrated trajectories and via RL for new trajectory discovery. Through simulation experiments, we show that (i) ACNMP enables the system to extrapolate to situations where pure LfD fails; (ii) Simultaneous training of the system through SL and RL preserves the shape of demonstrations while adapting to novel situations due to the shared representations used by both learners; (iii) ACNMP enables order-of-magnitude sample-efficient RL in extrapolation of reaching tasks compared to the existing approaches; (iv) ACNMPs can be used to implement skill transfer between robots having different morphology, with competitive learning speeds and importantly with less number of assumptions compared to the state-of-the-art approaches. Finally, we show the real-world suitability of ACNMPs through real robot experiments that involve obstacle avoidance, pick and place and pouring actions.},
keywords = {Deep Learning, movement primitives, Transfer Learning},
pubstate = {published},
tppubtype = {inproceedings}
}
To equip robots with dexterous skills, an effective approach is to first transfer the desired skill via Learning from Demonstration (LfD), then let the robot improve it by self-exploration via Reinforcement Learning (RL). In this paper, we propose a novel LfD+RL framework, namely Adaptive Conditional Neural Movement Primitives (ACNMP), that allows efficient policy improvement in novel environments and effective skill transfer between different agents. This is achieved through exploiting the latent representation learned by the underlying Conditional Neural Process (CNP) model, and simultaneous training of the model with supervised learning (SL) for acquiring the demonstrated trajectories and via RL for new trajectory discovery. Through simulation experiments, we show that (i) ACNMP enables the system to extrapolate to situations where pure LfD fails; (ii) Simultaneous training of the system through SL and RL preserves the shape of demonstrations while adapting to novel situations due to the shared representations used by both learners; (iii) ACNMP enables order-of-magnitude sample-efficient RL in extrapolation of reaching tasks compared to the existing approaches; (iv) ACNMPs can be used to implement skill transfer between robots having different morphology, with competitive learning speeds and importantly with less number of assumptions compared to the state-of-the-art approaches. Finally, we show the real-world suitability of ACNMPs through real robot experiments that involve obstacle avoidance, pick and place and pouring actions. | 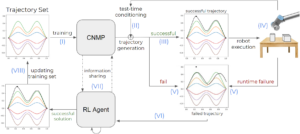 |
Rottmann, N.; Bruder, R.; Schweikard, A.; Rueckert, E. Exploiting Chlorophyll Fluorescense for Building Robust low-Cost Mowing Area Detectors Proceedings Article In: IEEE SENSORS , pp. 1–4, 2020. @inproceedings{Rottmann2020b,
title = {Exploiting Chlorophyll Fluorescense for Building Robust low-Cost Mowing Area Detectors},
author = {N. Rottmann and R. Bruder and A. Schweikard and E. Rueckert},
url = {https://cps.unileoben.ac.at/wp/IEEESensors2020Rottmann.pdf, Article File},
year = {2020},
date = {2020-10-26},
booktitle = {IEEE SENSORS },
journal = { IEEE SENSORS 2020 Conference, to be held from October 25-28, 2020},
pages = {1--4},
keywords = {mobile navigation, smart sensors},
pubstate = {published},
tppubtype = {inproceedings}
}
| 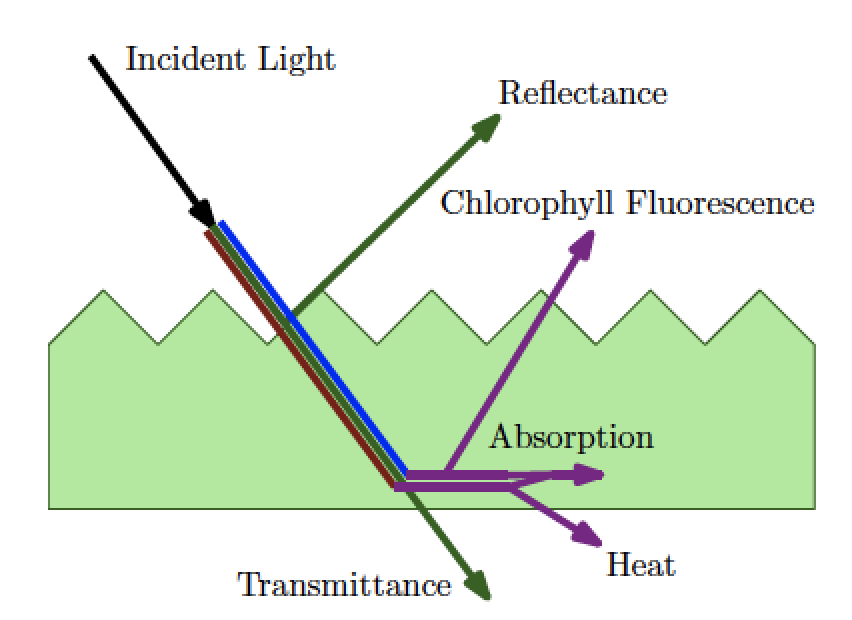 |
Rottmann, N.; Kunavar, T.; Babič, J.; Peters, J.; Rueckert, E. Learning Hierarchical Acquisition Functions for Bayesian Optimization Proceedings Article In: International Conference on Intelligent Robots and Systems (IROS’ 2020), 2020. @inproceedings{Rottmann2020HiBO,
title = {Learning Hierarchical Acquisition Functions for Bayesian Optimization},
author = {N. Rottmann and T. Kunavar and J. Babič and J. Peters and E. Rueckert},
url = {https://cps.unileoben.ac.at/wp/IROS2020Rottmann.pdf, Article File},
year = {2020},
date = {2020-10-25},
booktitle = {International Conference on Intelligent Robots and Systems (IROS’ 2020)},
keywords = {Reinforcement Learning},
pubstate = {published},
tppubtype = {inproceedings}
}
| 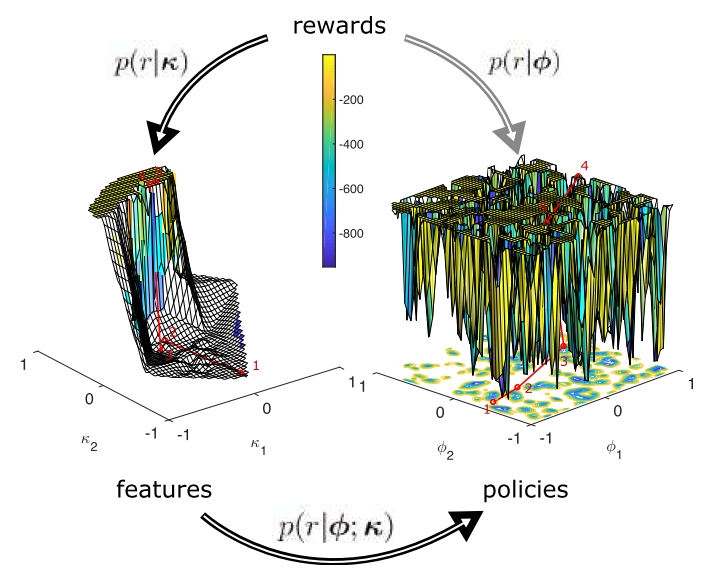 |
Rottmann, N.; Bruder, R.; Xue, H.; Schweikard, A.; Rueckert, E. Parameter Optimization for Loop Closure Detection in Closed Environments Proceedings Article In: Workshop Paper at the International Conference on Intelligent Robots and Systems (IROS), pp. 1–8, 2020. @inproceedings{Rottmann2020c,
title = {Parameter Optimization for Loop Closure Detection in Closed Environments},
author = {N. Rottmann and R. Bruder and H. Xue and A. Schweikard and E. Rueckert},
url = {https://cps.unileoben.ac.at/wp/IROSWS2020Rottmann.pdf, Article File},
year = {2020},
date = {2020-10-25},
booktitle = {Workshop Paper at the International Conference on Intelligent Robots and Systems (IROS)},
pages = {1--8},
keywords = {mobile navigation, Reinforcement Learning},
pubstate = {published},
tppubtype = {inproceedings}
}
| 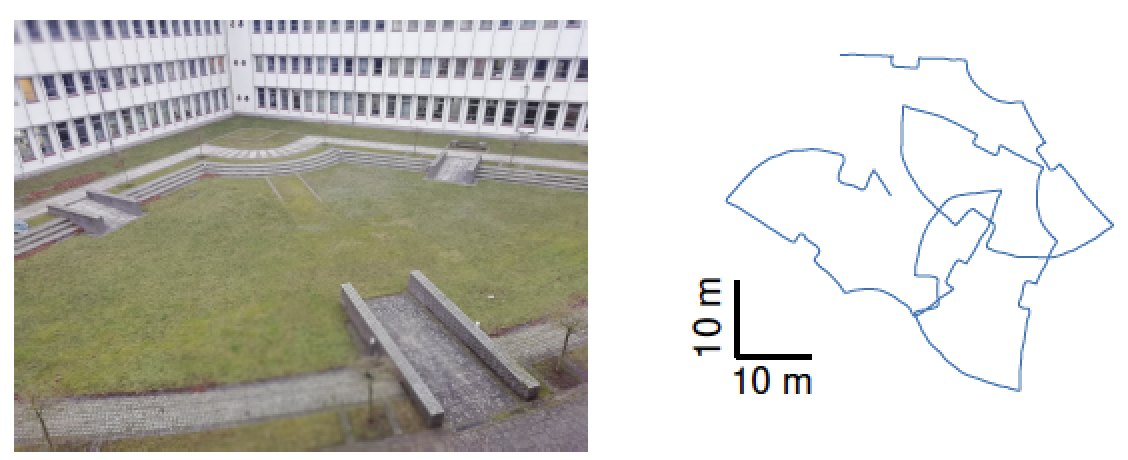 |
Rottmann, N.; Bruder, R.; Schweikard, A.; Rueckert, E. A novel Chlorophyll Fluorescence based approach for Mowing Area Classification Journal Article In: IEEE Sensors Journal, 2020. @article{Rottmann2020d,
title = {A novel Chlorophyll Fluorescence based approach for Mowing Area Classification},
author = {N. Rottmann and R. Bruder and A. Schweikard and E. Rueckert},
url = {https://cps.unileoben.ac.at/wp/IEEESensorsJournal2020Rottmann.pdf, Article File},
doi = {10.1109/JSEN.2020.3032722},
year = {2020},
date = {2020-10-12},
journal = {IEEE Sensors Journal},
keywords = {mobile navigation, smart sensors},
pubstate = {published},
tppubtype = {article}
}
| 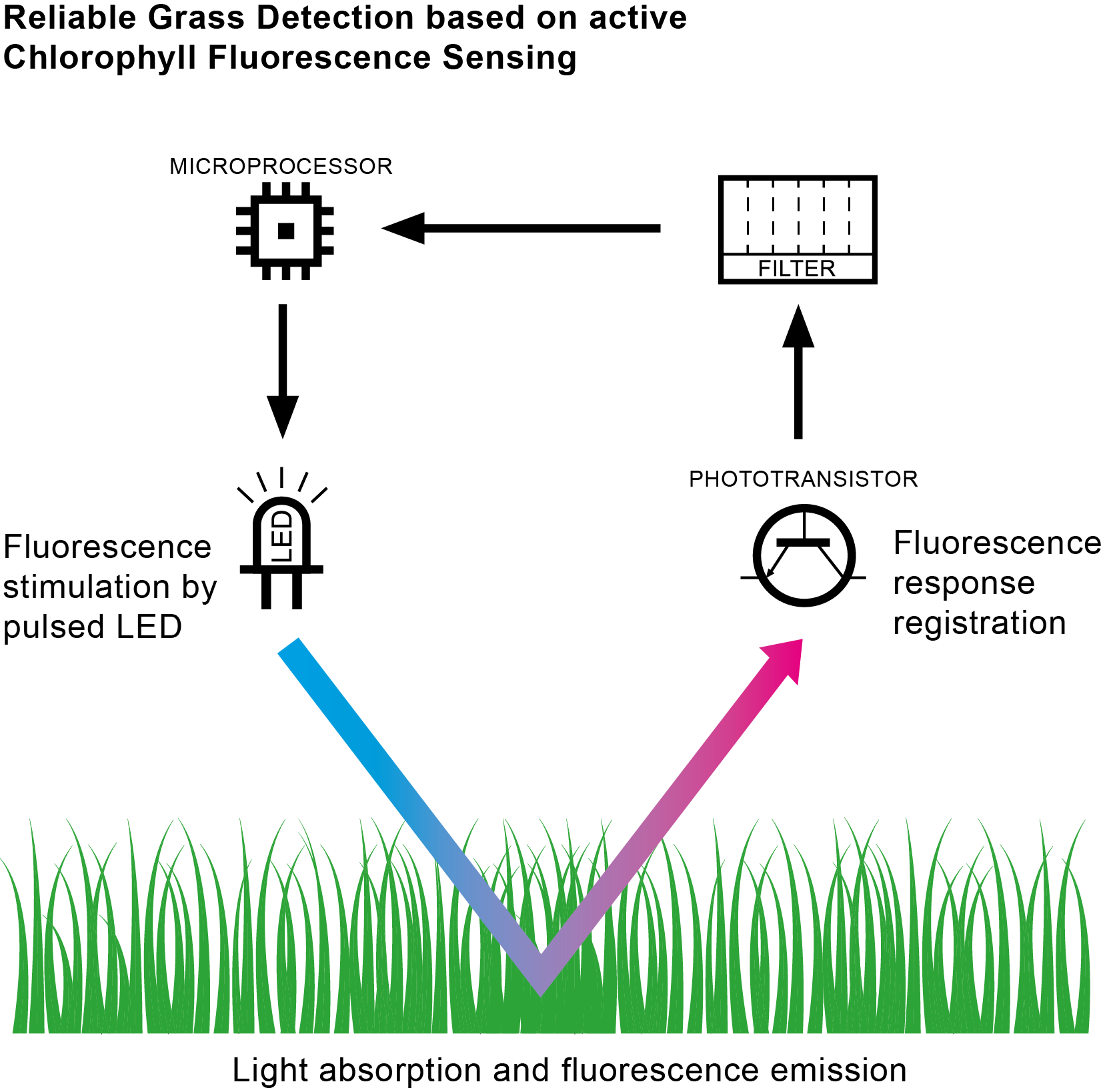 |
Tanneberg, Daniel; Rueckert, Elmar; Peters, Jan Evolutionary training and abstraction yields algorithmic generalization of neural computers Journal Article In: Nature Machine Intelligence, pp. 1–11, 2020. @article{Tanneberg2020,
title = {Evolutionary training and abstraction yields algorithmic generalization of neural computers},
author = {Daniel Tanneberg and Elmar Rueckert and Jan Peters },
url = {https://rdcu.be/caRlg, Article File},
doi = {10.1038/s42256-020-00255-1},
year = {2020},
date = {2020-10-10},
journal = {Nature Machine Intelligence},
pages = {1--11},
keywords = {neural network, Reinforcement Learning, Transfer Learning},
pubstate = {published},
tppubtype = {article}
}
| 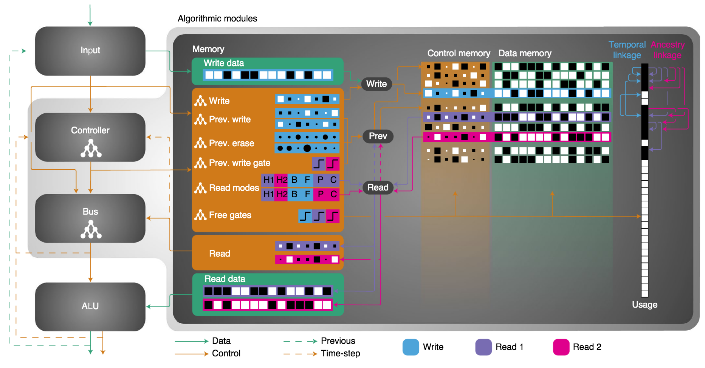 |
Tolga-Can Çallar, Elmar Rueckert; Böttger, Sven Efficient Body Registration Using Single-View Range Imaging and Generic Shape Templates Proceedings Article In: 54th Annual Conference of the German Society for Biomedical Engineering (BMT 2020), 2020. @inproceedings{Çallar2020,
title = {Efficient Body Registration Using Single-View Range Imaging and Generic Shape Templates},
author = {Tolga-Can Çallar, Elmar Rueckert and Sven Böttger},
url = {https://cps.unileoben.ac.at/wp/BMT2020Callar.pdf, Article File },
year = {2020},
date = {2020-09-20},
booktitle = {54th Annual Conference of the German Society for Biomedical Engineering (BMT 2020)},
journal = {Current Directions in Biomedical Engineering by De Gruyter},
keywords = {Medical Robotics},
pubstate = {published},
tppubtype = {inproceedings}
}
| 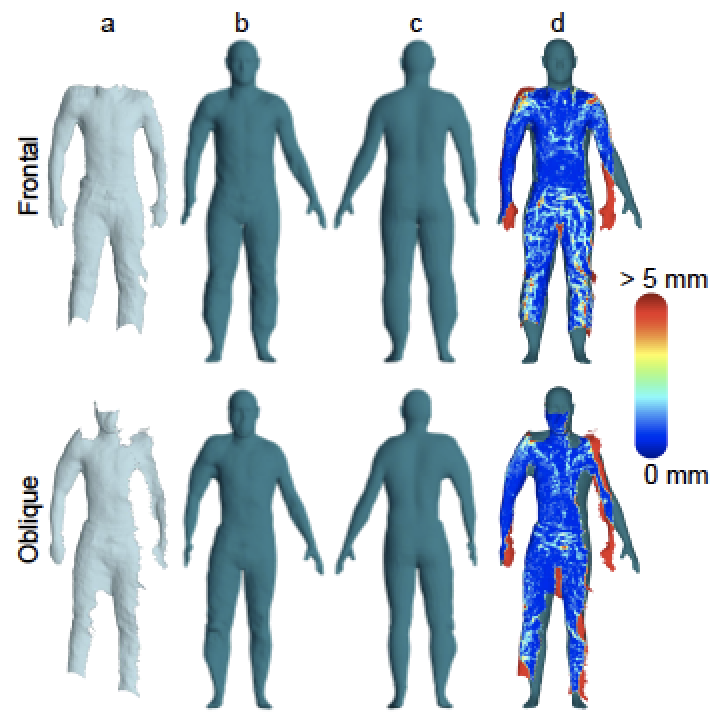 |
Xue, H.; Boettger, S.; Rottmann, N.; Pandya, H.; Bruder, R.; Neumann, G.; Schweikard, A.; Rueckert, E. Sample-Efficient Covariance Matrix Adaptation Evolutional Strategy via Simulated Rollouts in Neural Networks Proceedings Article In: International Conference on Advances in Signal Processing and Artificial Intelligence (ASPAI’ 2020), 2020. @inproceedings{Xue2020,
title = {Sample-Efficient Covariance Matrix Adaptation Evolutional Strategy via Simulated Rollouts in Neural Networks},
author = {H. Xue and S. Boettger and N. Rottmann and H. Pandya and R. Bruder and G. Neumann and A. Schweikard and E. Rueckert},
url = {https://cps.unileoben.ac.at/wp/ASPAI2020Xue.pdf, Article File},
year = {2020},
date = {2020-06-30},
booktitle = {International Conference on Advances in Signal Processing and Artificial Intelligence (ASPAI’ 2020)},
keywords = {Manipulation, Reinforcement Learning},
pubstate = {published},
tppubtype = {inproceedings}
}
| 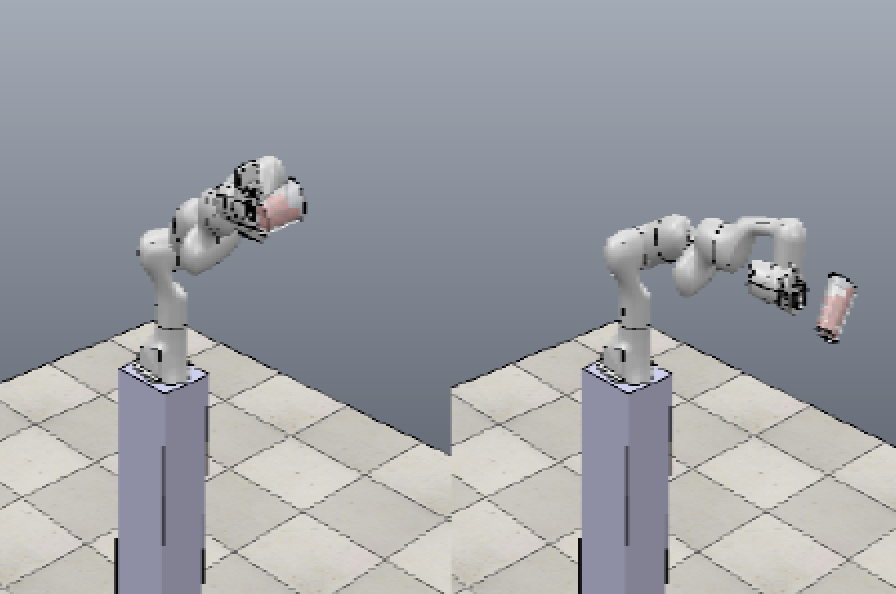 |
Cartoni, E.; Mannella, F.; Santucci, V. G.; Triesch, J.; Rueckert, E.; Baldassarre, G. REAL-2019: Robot open-Ended Autonomous Learning competition Journal Article In: Proceedings of Machine Learning Research, vol. 123, pp. 142-152, 2020, (NeurIPS 2019 Competition and Demonstration Track). @article{Cartoni2020,
title = {REAL-2019: Robot open-Ended Autonomous Learning competition},
author = {E. Cartoni and F. Mannella and V.G. Santucci and J. Triesch and E. Rueckert and G. Baldassarre},
editor = {H. J. Escalante and R. Hadsell},
url = {https://cps.unileoben.ac.at/wp/PMLR2020Cartoni.pdf, Article File},
year = {2020},
date = {2020-06-20},
journal = {Proceedings of Machine Learning Research},
volume = {123},
pages = {142-152},
note = {NeurIPS 2019 Competition and Demonstration Track},
keywords = {Manipulation, Simulation},
pubstate = {published},
tppubtype = {article}
}
| 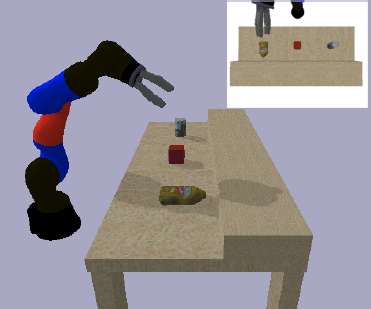 |
2019
|
Stark, Svenja; Peters, Jan; Rueckert, Elmar Experience Reuse with Probabilistic Movement Primitives Proceedings Article In: Proceedings of the IEEE/RSJ Conference on Intelligent Robots and Systems (IROS), 2019., 2019. @inproceedings{Stark2019,
title = {Experience Reuse with Probabilistic Movement Primitives},
author = {Svenja Stark and Jan Peters and Elmar Rueckert},
url = {https://cps.unileoben.ac.at/wp/IROS2019Stark.pdf, Article File},
year = {2019},
date = {2019-11-03},
booktitle = {Proceedings of the IEEE/RSJ Conference on Intelligent Robots and Systems (IROS), 2019.},
keywords = {movement primitives, Reinforcement Learning, Transfer Learning},
pubstate = {published},
tppubtype = {inproceedings}
}
| 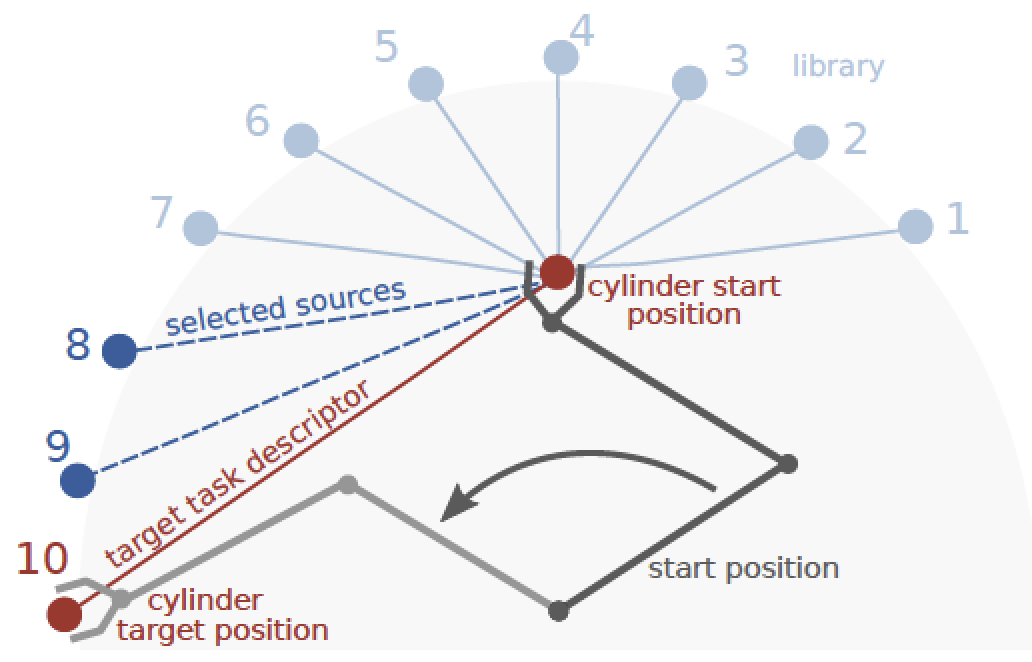 |
Boettger, S.; Callar, T. C.; Schweikard, A.; Rueckert, E. Medical robotics simulation framework for application-specific optimal kinematics Proceedings Article In: Current Directions in Biomedical Engineering 2019, pp. 1–5, 2019. @inproceedings{Boettger2019,
title = {Medical robotics simulation framework for application-specific optimal kinematics},
author = {S. Boettger and T.C. Callar and A. Schweikard and E. Rueckert},
url = {https://cps.unileoben.ac.at/wp/BMT2019Boettger.pdf, Article File},
year = {2019},
date = {2019-09-25},
booktitle = {Current Directions in Biomedical Engineering 2019},
pages = {1--5},
keywords = {Medical Robotics},
pubstate = {published},
tppubtype = {inproceedings}
}
| 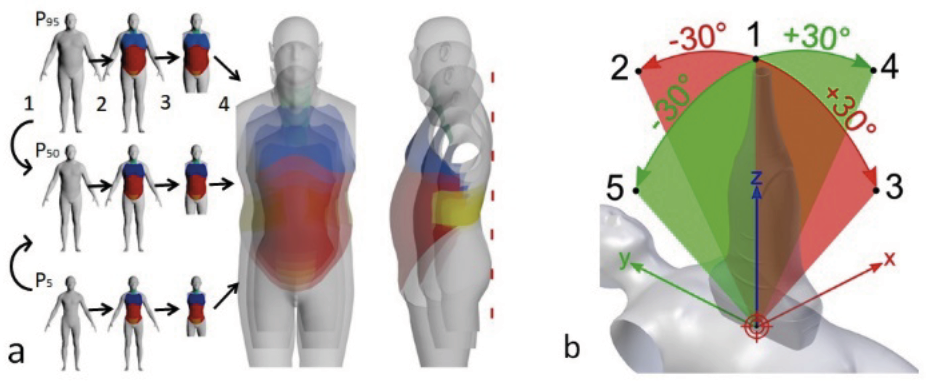 |
Rottmann, N.; Bruder, R.; Schweikard, A.; Rueckert, E. Loop Closure Detection in Closed Environments Proceedings Article In: European Conference on Mobile Robots (ECMR 2019), 2019, ISBN: 978-1-7281-3605-9. @inproceedings{Rottmann2019b,
title = {Loop Closure Detection in Closed Environments},
author = {N. Rottmann and R. Bruder and A. Schweikard and E. Rueckert},
url = {https://cps.unileoben.ac.at/wp/ECMR2019Rottmann.pdf, Article File},
isbn = {978-1-7281-3605-9},
year = {2019},
date = {2019-09-04},
booktitle = {European Conference on Mobile Robots (ECMR 2019)},
keywords = {mobile navigation},
pubstate = {published},
tppubtype = {inproceedings}
}
| 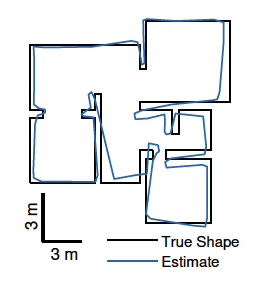 |
Rottmann, N.; Bruder, R.; Schweikard, A.; Rueckert, E. Cataglyphis ant navigation strategies solve the global localization problem in robots with binary sensors Proceedings Article In: Proceedings of International Conference on Bio-inspired Systems and Signal Processing (BIOSIGNALS), Prague, Czech Republic , 2019, ( February 22-24, 2019). @inproceedings{Rottmann2019,
title = {Cataglyphis ant navigation strategies solve the global localization problem in robots with binary sensors},
author = {N. Rottmann and R. Bruder and A. Schweikard and E. Rueckert},
url = {https://cps.unileoben.ac.at/wp/Biosignals2018Rottmann.pdf, Article File},
year = {2019},
date = {2019-02-22},
booktitle = {Proceedings of International Conference on Bio-inspired Systems and Signal Processing (BIOSIGNALS)},
address = {Prague, Czech Republic },
note = { February 22-24, 2019},
keywords = {constraint optimization, mobile navigation, Simulation},
pubstate = {published},
tppubtype = {inproceedings}
}
| 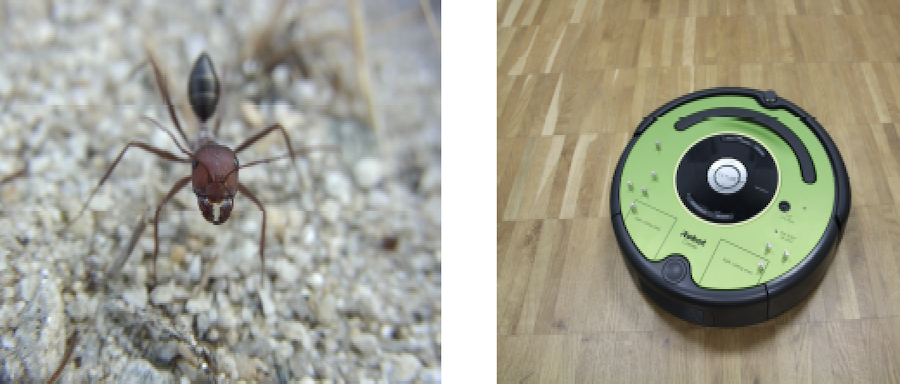 |
Rueckert, Elmar; Jauer, Philipp; Derksen, Alexander; Schweikard, Achim Dynamic Control Strategies for Cable-Driven Master Slave Robots Proceedings Article In: Keck, Tobias (Ed.): Proceedings on Minimally Invasive Surgery, Luebeck, Germany, 2019, (January 24-25, 2019). @inproceedings{Rueckert2019c,
title = {Dynamic Control Strategies for Cable-Driven Master Slave Robots},
author = {Elmar Rueckert and Philipp Jauer and Alexander Derksen and Achim Schweikard},
editor = {Tobias Keck},
doi = {10.18416/MIC.2019.1901007},
year = {2019},
date = {2019-01-24},
booktitle = {Proceedings on Minimally Invasive Surgery, Luebeck, Germany},
note = {January 24-25, 2019},
keywords = {Medical Robotics, Reinforcement Learning},
pubstate = {published},
tppubtype = {inproceedings}
}
| 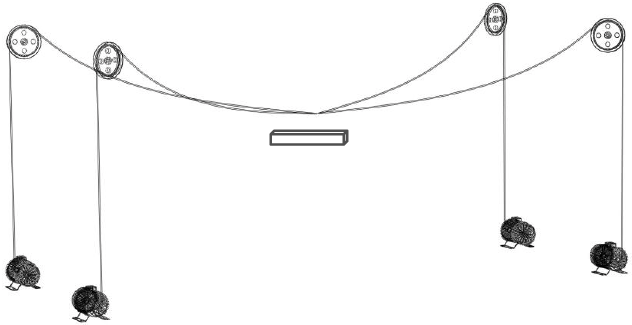 |
Tanneberg, Daniel; Peters, Jan; Rueckert, Elmar Intrinsic Motivation and Mental Replay enable Efficient Online Adaptation in Stochastic Recurrent Networks Journal Article In: Neural Networks - Elsevier, vol. 109, pp. 67-80, 2019, ISBN: 0893-6080, (Impact Factor of 7.197 (2017)). @article{Tanneberg2019,
title = {Intrinsic Motivation and Mental Replay enable Efficient Online Adaptation in Stochastic Recurrent Networks},
author = {Daniel Tanneberg and Jan Peters and Elmar Rueckert},
url = {https://cps.unileoben.ac.at/wp/NeuralNetworks2018Tanneberg.pdf, Article File},
doi = {10.1016/j.neunet.2018.10.005},
isbn = {0893-6080},
year = {2019},
date = {2019-01-01},
journal = {Neural Networks - Elsevier},
volume = {109},
pages = {67-80},
note = {Impact Factor of 7.197 (2017)},
keywords = {neural network, Probabilistic Inference, RNN, spiking},
pubstate = {published},
tppubtype = {article}
}
| 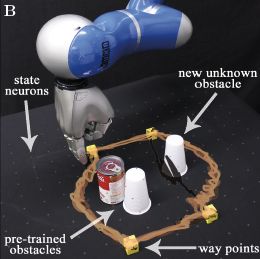 |
2018
|
Gondaliya, Kaushikkumar D.; Peters, Jan; Rueckert, Elmar Learning to Categorize Bug Reports with LSTM Networks Proceedings Article In: Proceedings of the International Conference on Advances in System Testing and Validation Lifecycle (VALID)., pp. 6, XPS (Xpert Publishing Services), Nice, France, 2018, ISBN: 978-1-61208-671-2, ( October 14-18, 2018). @inproceedings{Gondaliya2018,
title = {Learning to Categorize Bug Reports with LSTM Networks},
author = {Kaushikkumar D. Gondaliya and Jan Peters and Elmar Rueckert},
url = {https://cps.unileoben.ac.at/wp/VALID2018Gondaliya.pdf, Article File},
isbn = {978-1-61208-671-2},
year = {2018},
date = {2018-10-14},
booktitle = {Proceedings of the International Conference on Advances in System Testing and Validation Lifecycle (VALID).},
pages = {6},
publisher = {XPS (Xpert Publishing Services)},
address = {Nice, France},
note = { October 14-18, 2018},
keywords = {Natural Language Processing, neural network, RNN},
pubstate = {published},
tppubtype = {inproceedings}
}
| 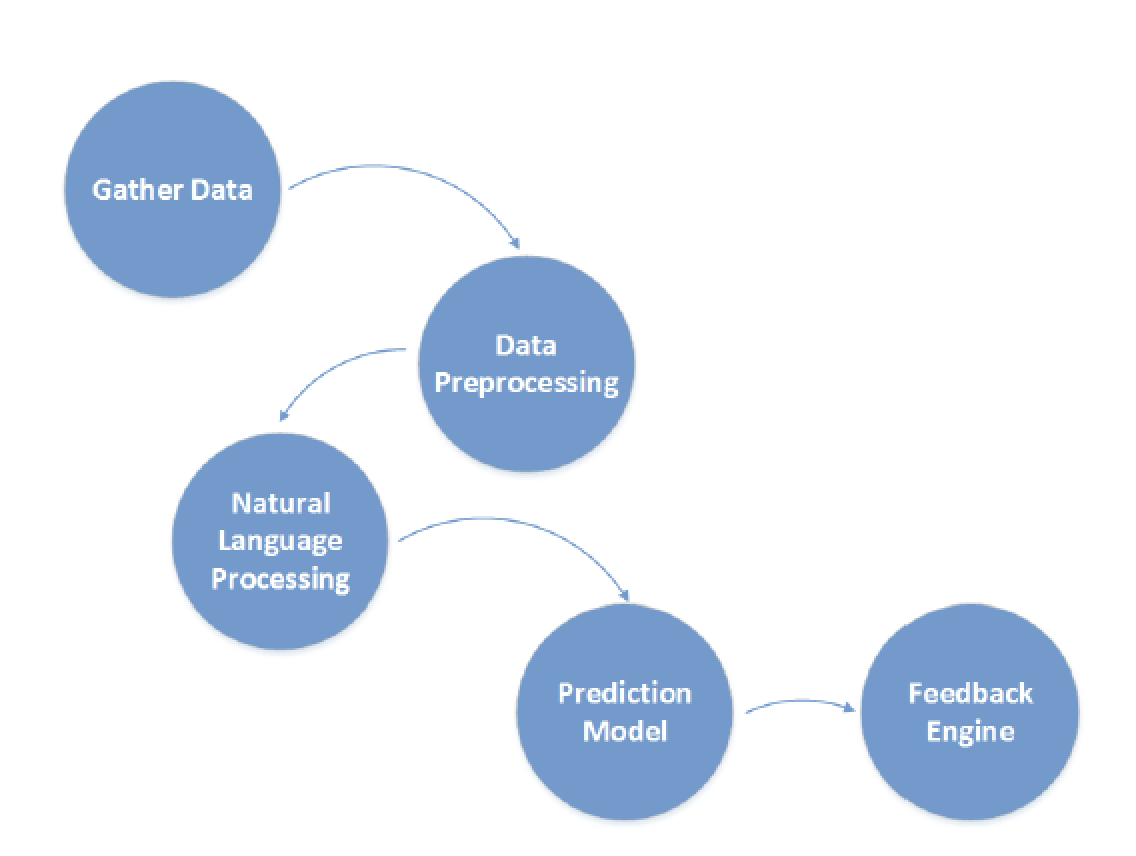 |
Sosic, Adrian; Zoubir, Abdelhak M.; Rueckert, Elmar; Peters, Jan; Koeppl, Heinz Inverse Reinforcement Learning via Nonparametric Spatio-Temporal Subgoal Modeling Journal Article In: Journal of Machine Learning Research (JMLR), vol. 19, no. 69, pp. 1-45, 2018. @article{Sosic2018,
title = {Inverse Reinforcement Learning via Nonparametric Spatio-Temporal Subgoal Modeling},
author = {Adrian Sosic and Abdelhak M. Zoubir and Elmar Rueckert and Jan Peters and
Heinz Koeppl},
url = {https://cps.unileoben.ac.at/wp/JMLR2018Sosic.pdf, Preprint Article File},
year = {2018},
date = {2018-10-08},
journal = {Journal of Machine Learning Research (JMLR)},
volume = {19},
number = {69},
pages = {1-45},
keywords = {movement primitives, Probabilistic Inference, Simulation},
pubstate = {published},
tppubtype = {article}
}
| 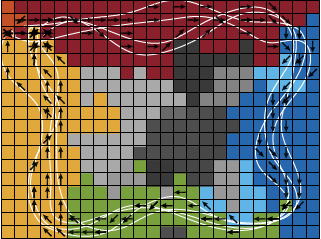 |
Paraschos, Alexandros; Rueckert, Elmar; Peters, Jan; Neumann, Gerhard Probabilistic Movement Primitives under Unknown System Dynamics Journal Article In: Advanced Robotics (ARJ), vol. 32, no. 6, pp. 297-310, 2018. @article{Paraschos2018,
title = {Probabilistic Movement Primitives under Unknown System Dynamics},
author = {Alexandros Paraschos and Elmar Rueckert and Jan Peters and Gerhard Neumann },
url = {https://cps.unileoben.ac.at/wp/AR2018Paraschos.pdf, Article File},
doi = {10.1080/01691864.2018.1437674},
year = {2018},
date = {2018-01-10},
journal = {Advanced Robotics (ARJ)},
volume = {32},
number = {6},
pages = {297-310},
keywords = {inverse dynamics, model learning, movement primitives},
pubstate = {published},
tppubtype = {article}
}
| 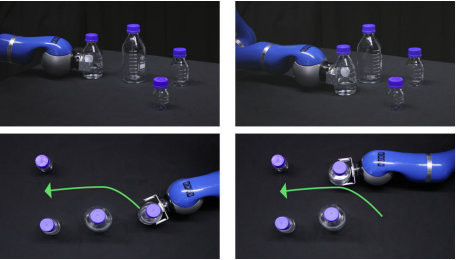 |
2017
|
Rueckert, Elmar; Nakatenus, Moritz; Tosatto, Samuele; Peters, Jan Learning Inverse Dynamics Models in O(n) time with LSTM networks Proceedings Article In: Proceedings of the International Conference on Humanoid Robots (HUMANOIDS), 2017. @inproceedings{Humanoids2017Rueckert,
title = {Learning Inverse Dynamics Models in O(n) time with LSTM networks},
author = {Elmar Rueckert and Moritz Nakatenus and Samuele Tosatto and Jan Peters},
url = {https://cps.unileoben.ac.at/wp/Humanoids2017Rueckert.pdf, Article File},
year = {2017},
date = {2017-11-15},
booktitle = {Proceedings of the International Conference on Humanoid Robots (HUMANOIDS)},
crossref = {p11091},
key = {goal-robots, skills4robots},
keywords = {inverse dynamics, model learning, RNN},
pubstate = {published},
tppubtype = {inproceedings}
}
| 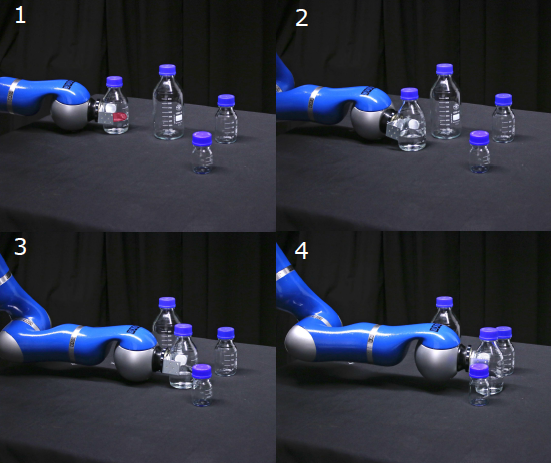 |
Tanneberg, Daniel; Peters, Jan; Rueckert, Elmar Efficient Online Adaptation with Stochastic Recurrent Neural Networks Proceedings Article In: Proceedings of the International Conference on Humanoid Robots (HUMANOIDS), 2017. @inproceedings{Tanneberg2017a,
title = {Efficient Online Adaptation with Stochastic Recurrent Neural Networks},
author = {Daniel Tanneberg and Jan Peters and Elmar Rueckert},
url = {https://cps.unileoben.ac.at/wp/Humanoids2017Tanneberg.pdf, Article File},
year = {2017},
date = {2017-11-15},
booktitle = {Proceedings of the International Conference on Humanoid Robots (HUMANOIDS)},
crossref = {p11092},
key = {goal-robots, skills4robots},
keywords = {intrinsic motivation, RNN, spiking},
pubstate = {published},
tppubtype = {inproceedings}
}
| 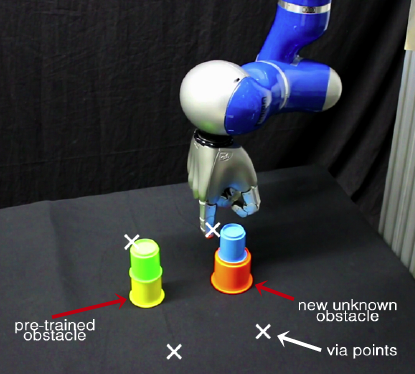 |
Stark, Svenja; Peters, Jan; Rueckert, Elmar A Comparison of Distance Measures for Learning Nonparametric Motor Skill Libraries Proceedings Article In: Proceedings of the International Conference on Humanoid Robots (HUMANOIDS), 2017. @inproceedings{Humanoids2017Stark,
title = {A Comparison of Distance Measures for Learning Nonparametric Motor Skill Libraries},
author = {Svenja Stark and Jan Peters and Elmar Rueckert},
url = {https://cps.unileoben.ac.at/wp/Humanoids2017Stark.pdf, Article File},
year = {2017},
date = {2017-11-15},
booktitle = {Proceedings of the International Conference on Humanoid Robots (HUMANOIDS)},
crossref = {p11093},
key = {goal-robots, skills4robots},
keywords = {intrinsic motivation, movement primitives},
pubstate = {published},
tppubtype = {inproceedings}
}
| 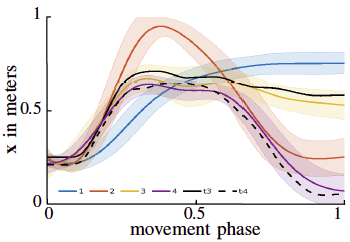 |
Thiem, Simon; Stark, Svenja; Tanneberg, Daniel; Peters, Jan; Rueckert, Elmar Simulation of the underactuated Sake Robotics Gripper in V-REP Proceedings Article In: Workshop at the International Conference on Humanoid Robots (HUMANOIDS), 2017. @inproceedings{Thiem2017b,
title = {Simulation of the underactuated Sake Robotics Gripper in V-REP},
author = {Simon Thiem and Svenja Stark and Daniel Tanneberg and Jan Peters and Elmar Rueckert},
url = {https://cps.unileoben.ac.at/wp/Humanoids2017Thiem.pdf},
year = {2017},
date = {2017-11-15},
booktitle = {Workshop at the International Conference on Humanoid Robots (HUMANOIDS)},
keywords = {Manipulation, Simulation},
pubstate = {published},
tppubtype = {inproceedings}
}
| 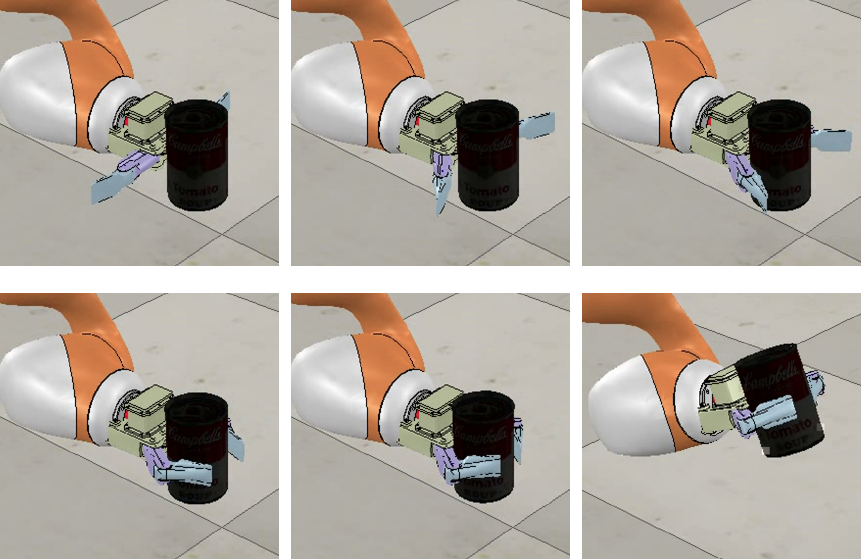 |
Tanneberg, Daniel; Peters, Jan; Rueckert, Elmar Online Learning with Stochastic Recurrent Neural Networks using Intrinsic Motivation Signals Proceedings Article In: Proceedings of the Conference on Robot Learning (CoRL), 2017. @inproceedings{Tanneberg2017,
title = {Online Learning with Stochastic Recurrent Neural Networks using Intrinsic Motivation Signals},
author = {Daniel Tanneberg and Jan Peters and Elmar Rueckert},
url = {https://cps.unileoben.ac.at/wp/CoRL2017Tanneberg.pdf, Article File},
year = {2017},
date = {2017-11-10},
booktitle = {Proceedings of the Conference on Robot Learning (CoRL)},
crossref = {p11088},
key = {goal-robots, skills4robots},
keywords = {intrinsic motivation, RNN, spiking},
pubstate = {published},
tppubtype = {inproceedings}
}
| 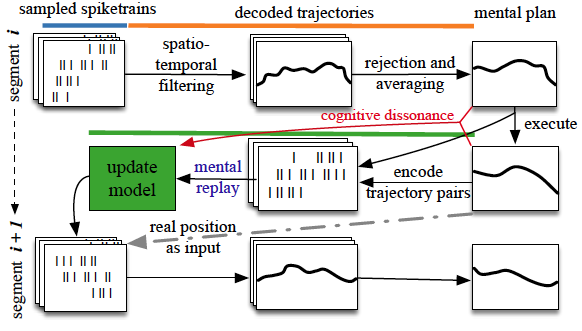 |
2016
|
Tanneberg, Daniel; Paraschos, Alexandros; Peters, Jan; Rueckert, Elmar Deep Spiking Networks for Model-based Planning in Humanoids Proceedings Article In: Proceedings of the International Conference on Humanoid Robots (HUMANOIDS), 2016. @inproceedings{tanneberg_humanoids16,
title = {Deep Spiking Networks for Model-based Planning in Humanoids},
author = {Daniel Tanneberg and Alexandros Paraschos and Jan Peters and Elmar Rueckert},
url = {https://cps.unileoben.ac.at/wp/Humanoids2016Tanneberg.pdf, Article File
https://cps.unileoben.ac.at/wp/resources/videos/humanoids_slower_540p.mp4, Supplementary Video},
year = {2016},
date = {2016-11-16},
booktitle = {Proceedings of the International Conference on Humanoid Robots (HUMANOIDS)},
crossref = {p10980},
key = {codyco and tacman},
keywords = {model learning, RNN, spiking},
pubstate = {published},
tppubtype = {inproceedings}
}
| 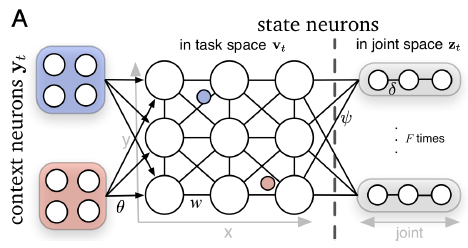 |
Azad, Morteza; Ortenzi, Valerio; Lin, Hsiu-Chin; Rueckert, Elmar; Mistry, Michael Model Estimation and Control of Complaint Contact Normal Force Proceedings Article In: Proceedings of the International Conference on Humanoid Robots (HUMANOIDS), 2016. @inproceedings{Humanoids2016Azad,
title = {Model Estimation and Control of Complaint Contact Normal Force},
author = {Morteza Azad and Valerio Ortenzi and Hsiu-Chin Lin and Elmar Rueckert and Michael Mistry},
url = {https://cps.unileoben.ac.at/wp/Humanoids2016Azad.pdf, Article File
https://cps.unileoben.ac.at/wp/resources/code/MATLAB_LocallyWeightedRegression_MEX_2015Rueckert.zip, MATLAB Code (fast LWR MEX-Function Implementation)},
year = {2016},
date = {2016-11-16},
booktitle = {Proceedings of the International Conference on Humanoid Robots (HUMANOIDS)},
crossref = {p10986},
key = {codyco},
keywords = {constraint optimization, human motor control, inverse dynamics, model learning},
pubstate = {published},
tppubtype = {inproceedings}
}
| 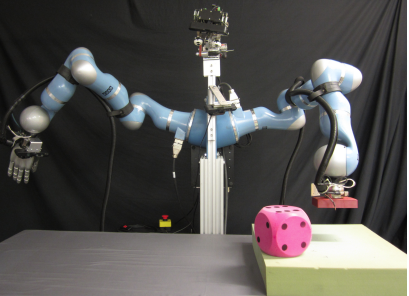 |
Rueckert, Elmar; Camernik, Jernej; Peters, Jan; Babic, Jan Probabilistic Movement Models Show that Postural Control Precedes and Predicts Volitional Motor Control Journal Article In: Nature Publishing Group: Scientific Reports, vol. 6, no. 28455, 2016. @article{Rueckert2016b,
title = {Probabilistic Movement Models Show that Postural Control Precedes and Predicts Volitional Motor Control},
author = {Elmar Rueckert and Jernej Camernik and Jan Peters and Jan Babic},
url = {https://cps.unileoben.ac.at/wp/SciReps_HumanContacts.pdf, Article File
https://cps.unileoben.ac.at/wp/resources/code/MATLAB_ProbabilisticTrajectoryModel_2016Rueckert.zip, MATLAB Code
https://cps.unileoben.ac.at/wp/SciReps_HumanContacts_Supplement.pdf, Supplement},
doi = {10.1038/srep28455},
year = {2016},
date = {2016-06-02},
journal = {Nature Publishing Group: Scientific Reports},
volume = {6},
number = {28455},
keywords = {human motor control, muscle synergies, postural control},
pubstate = {published},
tppubtype = {article}
}
| 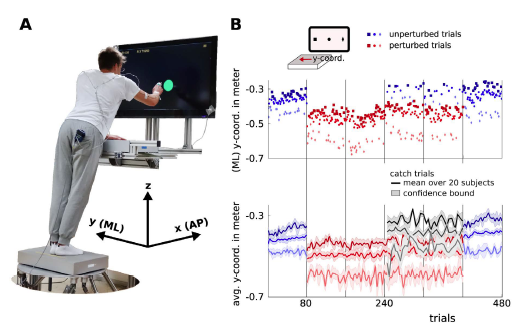 |
Rueckert, Elmar; Kappel, David; Tanneberg, Daniel; Pecevski, Dejan; Peters, Jan Recurrent Spiking Networks Solve Planning Tasks Journal Article In: Nature Publishing Group: Scientific Reports, vol. 6, no. 21142, 2016. @article{Rueckert2016a,
title = {Recurrent Spiking Networks Solve Planning Tasks},
author = {Elmar Rueckert and David Kappel and Daniel Tanneberg and Dejan Pecevski and Jan Peters},
url = {https://cps.unileoben.ac.at/wp/SciReps_NeuralPlanning.pdf, Article File
https://cps.unileoben.ac.at/wp/SciReps_NeuralPlanning_Supplement.pdf, Supplement
https://cps.unileoben.ac.at/wp/resources/code/MATLAB_SpikingNeuralPlanning_2016Rueckert.zip, MATLAB Code},
doi = {10.1038/srep21142},
year = {2016},
date = {2016-01-15},
journal = {Nature Publishing Group: Scientific Reports},
volume = {6},
number = {21142},
keywords = {RNN, spiking},
pubstate = {published},
tppubtype = {article}
}
| 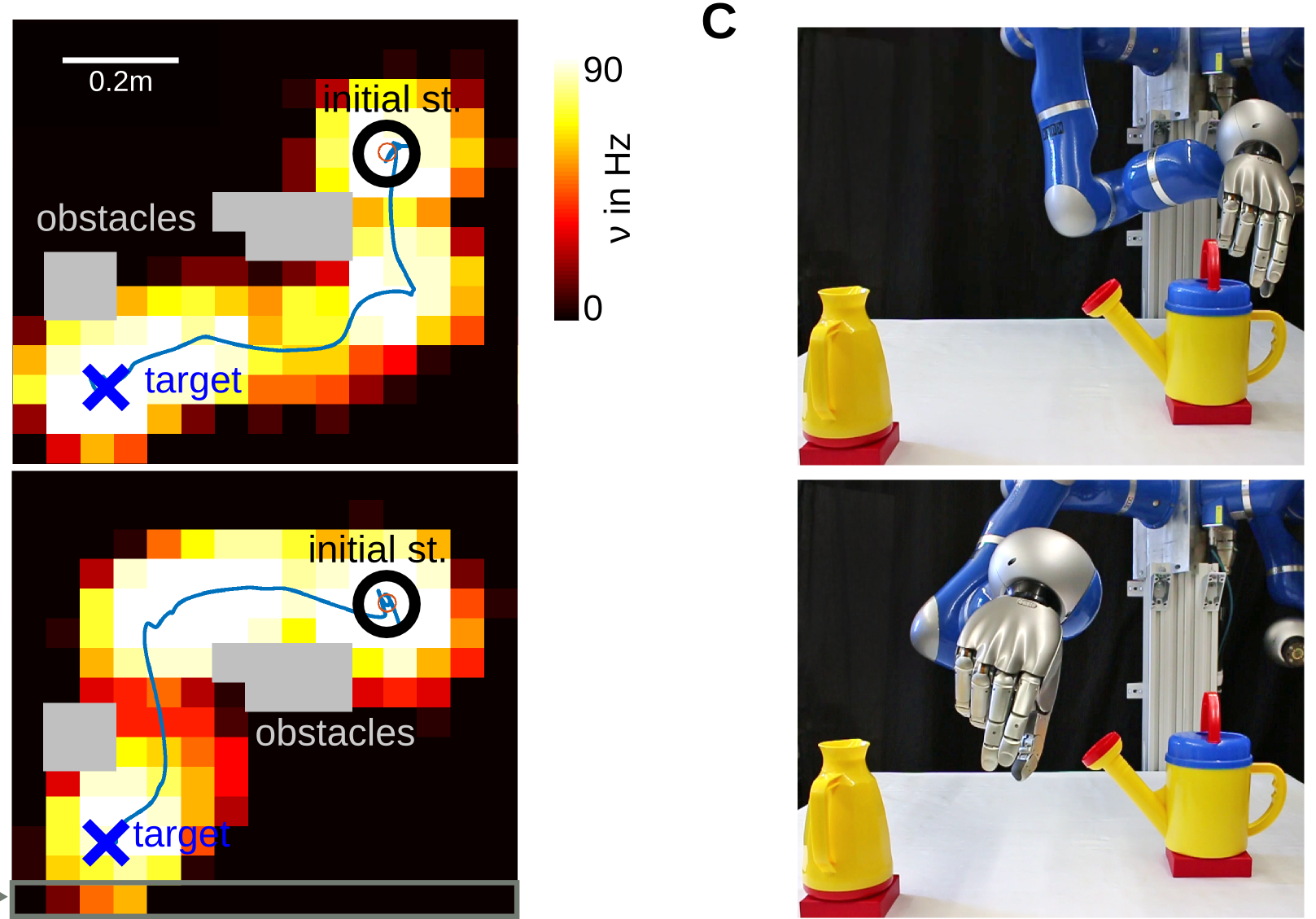 |
Kohlschuetter, Jan; Peters, Jan; Rueckert, Elmar Learning Probabilistic Features from EMG Data for Predicting Knee Abnormalities Proceedings Article In: Proceedings of the XIV Mediterranean Conference on Medical and Biological Engineering and Computing (MEDICON), 2016. @inproceedings{Kohlschuetter2016,
title = {Learning Probabilistic Features from EMG Data for Predicting Knee Abnormalities},
author = {Jan Kohlschuetter and Jan Peters and Elmar Rueckert},
url = {https://cps.unileoben.ac.at/wp/KohlschuetterMEDICON_2016.pdf, Article File},
year = {2016},
date = {2016-01-01},
booktitle = {Proceedings of the XIV Mediterranean Conference on Medical and Biological Engineering and Computing (MEDICON)},
crossref = {p10898},
key = {codyco, tacman},
keywords = {graphical models, muscle synergies},
pubstate = {published},
tppubtype = {inproceedings}
}
| 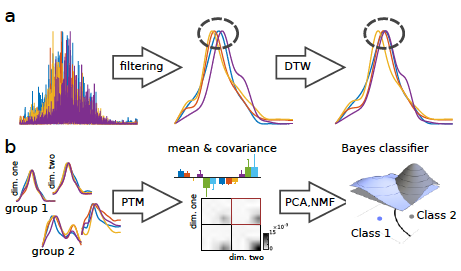 |
Modugno, Valerio; Neumann, Gerhard; Rueckert, Elmar; Oriolo, Giuseppe; Peters, Jan; Ivaldi, Serena Learning soft task priorities for control of redundant robots Proceedings Article In: Proceedings of the International Conference on Robotics and Automation (ICRA), 2016. @inproceedings{Modugno_PICRA_2016,
title = {Learning soft task priorities for control of redundant robots},
author = {Valerio Modugno and Gerhard Neumann and Elmar Rueckert and Giuseppe Oriolo and Jan Peters and Serena Ivaldi},
url = {https://cps.unileoben.ac.at/wp/ICRA2016Modugno.pdf, Article File},
year = {2016},
date = {2016-01-01},
booktitle = {Proceedings of the International Conference on Robotics and Automation (ICRA)},
crossref = {p10900},
key = {codyco},
keywords = {constraint optimization, policy search},
pubstate = {published},
tppubtype = {inproceedings}
}
| 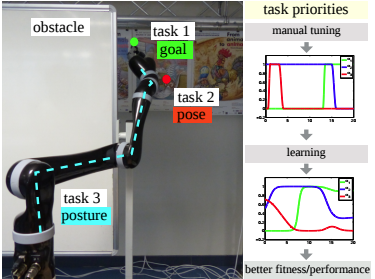 |
Sharma, David; Tanneberg, Daniel; Grosse-Wentrup, Moritz; Peters, Jan; Rueckert, Elmar Adaptive Training Strategies for BCIs Proceedings Article In: Cybathlon Symposium, 2016. @inproceedings{Sharma2016,
title = {Adaptive Training Strategies for BCIs},
author = {David Sharma and Daniel Tanneberg and Moritz Grosse-Wentrup and Jan Peters and Elmar Rueckert},
url = {https://cps.unileoben.ac.at/wp/Cybathlon2016Sharma.pdf, Article File},
year = {2016},
date = {2016-01-01},
booktitle = {Cybathlon Symposium},
crossref = {p10952},
keywords = {human motor control, Reinforcement Learning},
pubstate = {published},
tppubtype = {inproceedings}
}
| 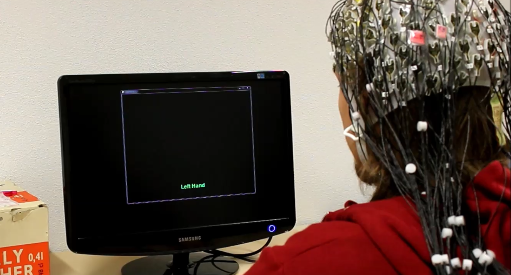 |
Weber, Paul; Rueckert, Elmar; Calandra, Roberto; Peters, Jan; Beckerle, Philipp A Low-cost Sensor Glove with Vibrotactile Feedback and Multiple Finger Joint and Hand Motion Sensing for Human-Robot Interaction Proceedings Article In: Proceedings of the IEEE International Symposium on Robot and Human Interactive Communication (RO-MAN), 2016. @inproceedings{ROMANS16_daglove,
title = {A Low-cost Sensor Glove with Vibrotactile Feedback and Multiple Finger Joint and Hand Motion Sensing for Human-Robot Interaction},
author = {Paul Weber and Elmar Rueckert and Roberto Calandra and Jan Peters and Philipp Beckerle},
url = {https://cps.unileoben.ac.at/wp/ROMANS2016Weber.pdf, Article File
https://cps.unileoben.ac.at/wp/resources/code/MATLAB_Arduino_SensorGlove_2015Rueckert.zip, MATLAB Code (JAVA Interface), ARDUINO Firmware
https://cps.unileoben.ac.at/wp/resources/code/MATLAB_SensorGloveMexInterface_2015Rueckert.zip, MATLAB Code (MEX-Function Demo Interface)},
year = {2016},
date = {2016-01-01},
booktitle = {Proceedings of the IEEE International Symposium on Robot and Human Interactive Communication (RO-MAN)},
crossref = {p10949},
key = {codyco},
keywords = {graphical models},
pubstate = {published},
tppubtype = {inproceedings}
}
| 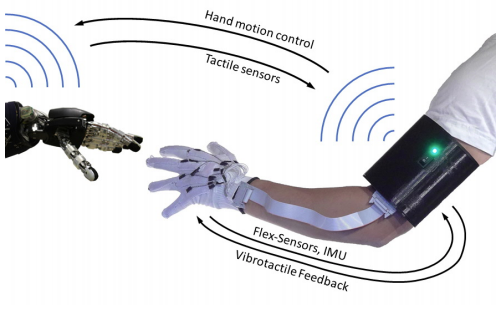 |
2015
|
Calandra, Roberto; Ivaldi, Serena; Deisenroth, Marc; Rueckert, Elmar; Peters, Jan Learning Inverse Dynamics Models with Contacts Proceedings Article In: Proceedings of the International Conference on Robotics and Automation (ICRA), 2015. @inproceedings{Calandra2015,
title = {Learning Inverse Dynamics Models with Contacts},
author = {Roberto Calandra and Serena Ivaldi and Marc Deisenroth and Elmar Rueckert and Jan Peters},
url = {https://cps.unileoben.ac.at/wp/ICRA15Calandra.pdf, Article File},
year = {2015},
date = {2015-01-01},
booktitle = {Proceedings of the International Conference on Robotics and Automation (ICRA)},
crossref = {p10794},
key = {codyco},
keywords = {inverse dynamics, model learning, neural network},
pubstate = {published},
tppubtype = {inproceedings}
}
| 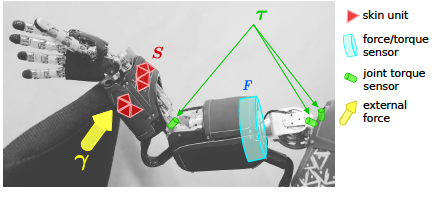 |
Rueckert, Elmar; Mundo, Jan; Paraschos, Alexandros; Peters, Jan; Neumann, Gerhard Extracting Low-Dimensional Control Variables for Movement Primitives Proceedings Article In: Proceedings of the International Conference on Robotics and Automation (ICRA), 2015. @inproceedings{Rueckert2015,
title = {Extracting Low-Dimensional Control Variables for Movement Primitives},
author = {Elmar Rueckert and Jan Mundo and Alexandros Paraschos and Jan Peters and Gerhard Neumann},
url = {https://cps.unileoben.ac.at/wp/ICRA2015Rueckert.pdf, Article File
https://cps.unileoben.ac.at/wp/resources/code/MATLAB_LatentManifoldPrimitives_2015Rueckert.zip, MATALB Code
https://cps.unileoben.ac.at/wp/resources/videos/KUKA_LatentManifold_ProMPs_REAL_Elmar.mp4, Supplementary Video},
year = {2015},
date = {2015-01-01},
booktitle = {Proceedings of the International Conference on Robotics and Automation (ICRA)},
crossref = {p10796},
key = {3rdhand, codyco},
keywords = {movement primitives, Probabilistic Inference},
pubstate = {published},
tppubtype = {inproceedings}
}
| 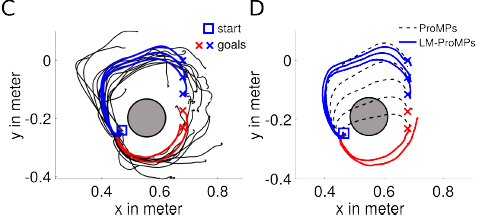 |
Paraschos, Alexandros; Rueckert, Elmar; Peters, Jan; Neumann, Gerhard Model-Free Probabilistic Movement Primitives for Physical Interaction Proceedings Article In: Proceedings of the IEEE/RSJ Conference on Intelligent Robots and Systems (IROS), 2015. @inproceedings{Paraschos2015,
title = {Model-Free Probabilistic Movement Primitives for Physical Interaction},
author = {Alexandros Paraschos and Elmar Rueckert and Jan Peters and Gerhard Neumann},
url = {https://cps.unileoben.ac.at/wp/IROS2015Paraschos.pdf, Article File},
year = {2015},
date = {2015-01-01},
booktitle = {Proceedings of the IEEE/RSJ Conference on Intelligent Robots and Systems (IROS)},
crossref = {p10832},
key = {codyco},
keywords = {inverse dynamics, movement primitives},
pubstate = {published},
tppubtype = {inproceedings}
}
| 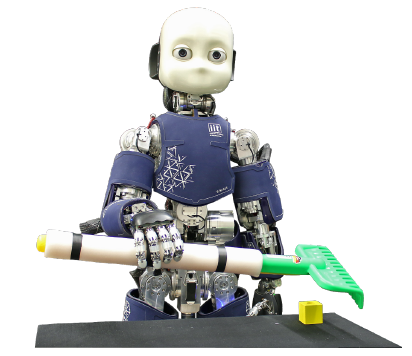 |
Rueckert, Elmar; Lioutikov, Rudolf; Calandra, Roberto; Schmidt, Marius; Beckerle, Philipp; Peters, Jan Low-cost Sensor Glove with Force Feedback for Learning from Demonstrations using Probabilistic Trajectory Representations Proceedings Article In: ICRA 2015 Workshop on Tactile and force sensing for autonomous compliant intelligent robots, 2015. @inproceedings{Rueckert2015b,
title = {Low-cost Sensor Glove with Force Feedback for Learning from Demonstrations using Probabilistic Trajectory Representations},
author = {Elmar Rueckert and Rudolf Lioutikov and Roberto Calandra and Marius Schmidt and Philipp Beckerle and Jan Peters},
url = {https://cps.unileoben.ac.at/wp/ICRA2015Rueckertb.pdf, Article File
https://cps.unileoben.ac.at/wp/resources/code/MATLAB_Arduino_SensorGlove_2015Rueckert.zip, MATLAB Code (JAVA Interface), ARDUINO Firmware
https://cps.unileoben.ac.at/wp/resources/code/MATLAB_SensorGloveMexInterface_2015Rueckert.zip, MATLAB Code (MEX-Function Demo Interface)},
year = {2015},
date = {2015-01-01},
booktitle = {ICRA 2015 Workshop on Tactile and force sensing for autonomous compliant intelligent robots},
crossref = {p10831},
key = {codyco},
keywords = {graphical models},
pubstate = {published},
tppubtype = {inproceedings}
}
| 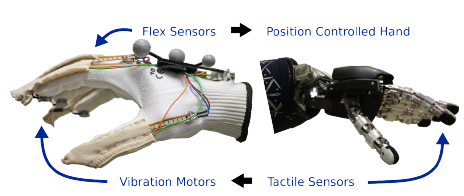 |
2014
|
Rueckert, Elmar Biologically inspired motor skill learning in robotics through probabilistic inference PhD Thesis Technical University Graz, 2014. @phdthesis{Rueckert2014a,
title = {Biologically inspired motor skill learning in robotics through probabilistic inference},
author = {Elmar Rueckert},
url = {https://cps.unileoben.ac.at/wp/PhDThesis2014Rueckert.pdf, Article File},
year = {2014},
date = {2014-02-04},
school = {Technical University Graz},
keywords = {graphical models, locomotion, model learning, morphological compuation, movement primitives, policy search, postural control, Probabilistic Inference, Reinforcement Learning, RNN, SOC, spiking},
pubstate = {published},
tppubtype = {phdthesis}
}
| 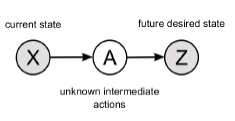 |
Rueckert, Elmar; Mindt, Max; Peters, Jan; Neumann, Gerhard Robust Policy Updates for Stochastic Optimal Control Proceedings Article In: Proceedings of the International Conference on Humanoid Robots (HUMANOIDS), 2014. @inproceedings{Rueckert2014,
title = {Robust Policy Updates for Stochastic Optimal Control},
author = {Elmar Rueckert and Max Mindt and Jan Peters and Gerhard Neumann},
url = {https://cps.unileoben.ac.at/wp/Humanoids2014Rueckert.pdf, Article File
https://cps.unileoben.ac.at/wp/resources/code/MATLAB_RobustStochasticOptimalControl_2015Rueckert.zip, MATLAB Code},
year = {2014},
date = {2014-01-01},
booktitle = {Proceedings of the International Conference on Humanoid Robots (HUMANOIDS)},
crossref = {p10768},
key = {codyco},
keywords = {policy search, Probabilistic Inference, SOC},
pubstate = {published},
tppubtype = {inproceedings}
}
| 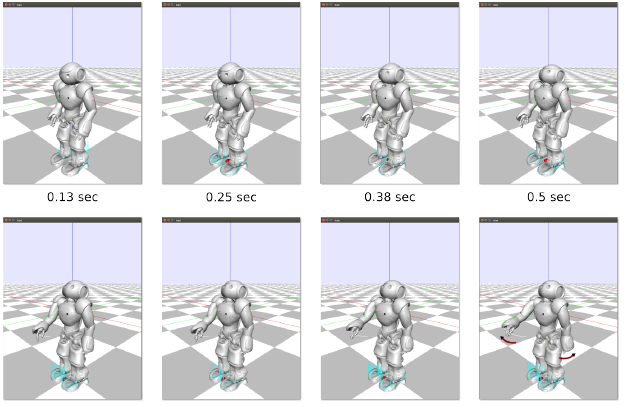 |
2013
|
Rueckert, Elmar; d'Avella, Andrea Learned parametrized dynamic movement primitives with shared synergies for controlling robotic and musculoskeletal systems Journal Article In: Frontiers in Computational Neuroscience, vol. 7, no. 138, 2013. @article{Rueckert2013b,
title = {Learned parametrized dynamic movement primitives with shared synergies for controlling robotic and musculoskeletal systems},
author = {Elmar Rueckert and Andrea d'Avella},
url = {https://cps.unileoben.ac.at/wp/Frontiers2013bRueckert.pdf, Article File
https://cps.unileoben.ac.at/wp/resources/code/MATLAB_PlanarWalkerSimulator_2013Rueckert.zip, MATLAB Code (Planar Walker Simulator)
https://cps.unileoben.ac.at/wp/resources/code/MATLAB_OpenSimMEXInterface_2013Rueckert.zip, MATLAB Code (OpenSim MEX-Function Interface)},
doi = {10.3389/fncom.2013.00138},
year = {2013},
date = {2013-10-17},
journal = {Frontiers in Computational Neuroscience},
volume = {7},
number = {138},
keywords = {locomotion, movement primitives, muscle synergies},
pubstate = {published},
tppubtype = {article}
}
| 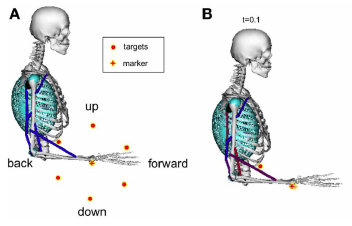 |
Rueckert, Elmar; Neumann, Gerhard; Toussaint, Marc; Maass, Wolfgang Learned graphical models for probabilistic planning provide a new class of movement primitives Journal Article In: Frontiers in Computational Neuroscience, vol. 6, no. 97, 2013. @article{Rueckert2013,
title = { Learned graphical models for probabilistic planning provide a new class of movement primitives},
author = {Elmar Rueckert and Gerhard Neumann and Marc Toussaint and Wolfgang Maass},
url = {https://cps.unileoben.ac.at/wp/Frontiers2013aRueckert.pdf, Article File},
doi = {10.3389/fncom.2012.00097},
year = {2013},
date = {2013-01-02},
journal = {Frontiers in Computational Neuroscience},
volume = {6},
number = {97},
keywords = {graphical models, movement primitives, Probabilistic Inference},
pubstate = {published},
tppubtype = {article}
}
| 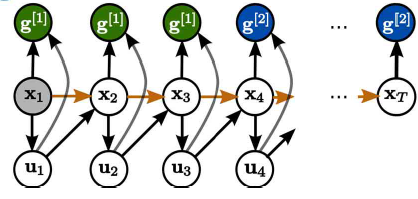 |
Rueckert, Elmar; d'Avella, Andrea Learned Muscle Synergies as Prior in Dynamical Systems for Controlling Bio-mechanical and Robotic Systems Proceedings Article In: Abstracts of Neural Control of Movement Conference (NCM), Conference Talk, pp. 27–28, 2013. @inproceedings{Rueckert2013,
title = {Learned Muscle Synergies as Prior in Dynamical Systems for Controlling Bio-mechanical and Robotic Systems},
author = {Elmar Rueckert and Andrea d'Avella},
url = {https://cps.unileoben.ac.at/wp/Frontiers2013bRueckert.pdf, Article File},
year = {2013},
date = {2013-01-01},
booktitle = {Abstracts of Neural Control of Movement Conference (NCM), Conference Talk},
pages = {27--28},
crossref = {p10682},
keywords = {muscle synergies, policy search, Reinforcement Learning},
pubstate = {published},
tppubtype = {inproceedings}
}
| 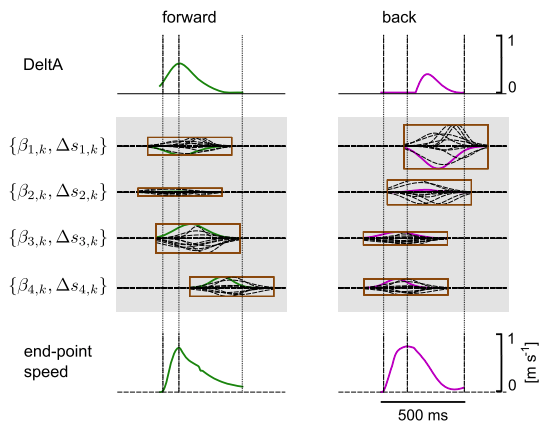 |
2012
|
Rueckert, Elmar; Neumann, Gerhard Stochastic Optimal Control Methods for Investigating the Power of Morphological Computation Journal Article In: Artificial Life, vol. 19, no. 1, 2012. @article{Rueckert2012,
title = {Stochastic Optimal Control Methods for Investigating the Power of Morphological Computation},
author = {Elmar Rueckert and Gerhard Neumann},
url = {https://cps.unileoben.ac.at/wp/ArtificialLife2012Rueckert.pdf, Article File},
doi = {10.1162/ARTL_a_00085},
year = {2012},
date = {2012-11-27},
journal = {Artificial Life},
volume = {19},
number = {1},
keywords = {morphological compuation, Probabilistic Inference, SOC},
pubstate = {published},
tppubtype = {article}
}
| 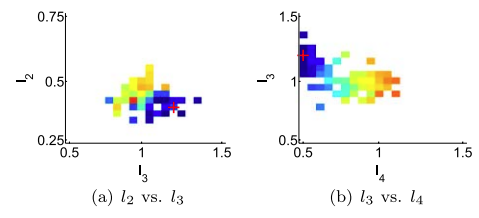 |
2011
|
Rueckert, Elmar; Neumann, Gerhard A study of Morphological Computation by using Probabilistic Inference for Motor Planning Proceedings Article In: Proceedings of the 2nd International Conference on Morphological Computation (ICMC), pp. 51–53, 2011. @inproceedings{Rueckert2011,
title = {A study of Morphological Computation by using Probabilistic Inference for Motor Planning},
author = {Elmar Rueckert and Gerhard Neumann},
url = {https://cps.unileoben.ac.at/wp/ICMC2011Rueckert.pdf, Article File},
year = {2011},
date = {2011-01-01},
booktitle = {Proceedings of the 2nd International Conference on Morphological Computation (ICMC)},
pages = {51--53},
crossref = {p10680},
keywords = {graphical models, morphological compuation, Probabilistic Inference, SOC},
pubstate = {published},
tppubtype = {inproceedings}
}
| 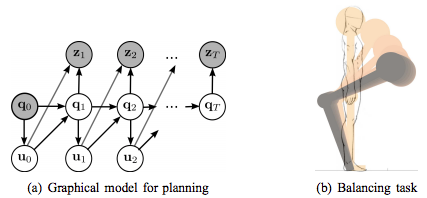 |
2010
|
Rueckert, Elmar Simultaneous localisation and mapping for mobile robots with recent sensor technologies Masters Thesis Technical University Graz, 2010. @mastersthesis{Rueckert2010,
title = {Simultaneous localisation and mapping for mobile robots with recent sensor technologies},
author = {Elmar Rueckert},
url = {https://cps.unileoben.ac.at/wp/MScThesis2009Rueckert.pdf, Article File},
year = {2010},
date = {2010-01-28},
school = {Technical University Graz},
keywords = {Probabilistic Inference},
pubstate = {published},
tppubtype = {mastersthesis}
}
| 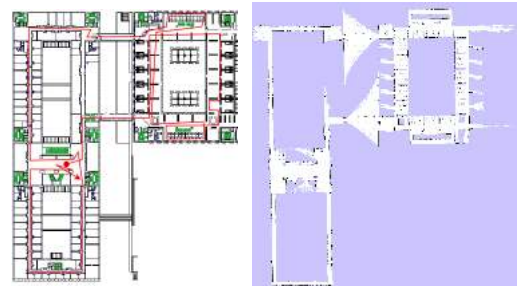 |